The 18 best data management tools for your organization
‘Data’ has become somewhat of a buzzword. Many organizations are aware of its importance in making better business decisions, but aren’t necessarily managing it in the right way.
A good data management strategy should deliver a single source of truth that can be relied upon and accessed by different teams across an organization, regardless of their technical ability.
And to reach this point, companies need to get the best data management tools in place. In this post, we’ll list out what we believe these are, covering everything from behavioral data tools to warehousing and reverse ETL. While this list is by no means exhaustive, we hope it will provide a solid starting point to build a data-informed culture at your organization.
Behavioral data management tools
At a general level, behavioral data describes the interaction between your customers and your organisation. This interaction (or ‘event’) can occur on a website, app, or any other interface that can be tracked. A behavioral data platform helps to collect, validate, and model these events so multiple stakeholders can get the maximum value from the data.
1. Snowplow
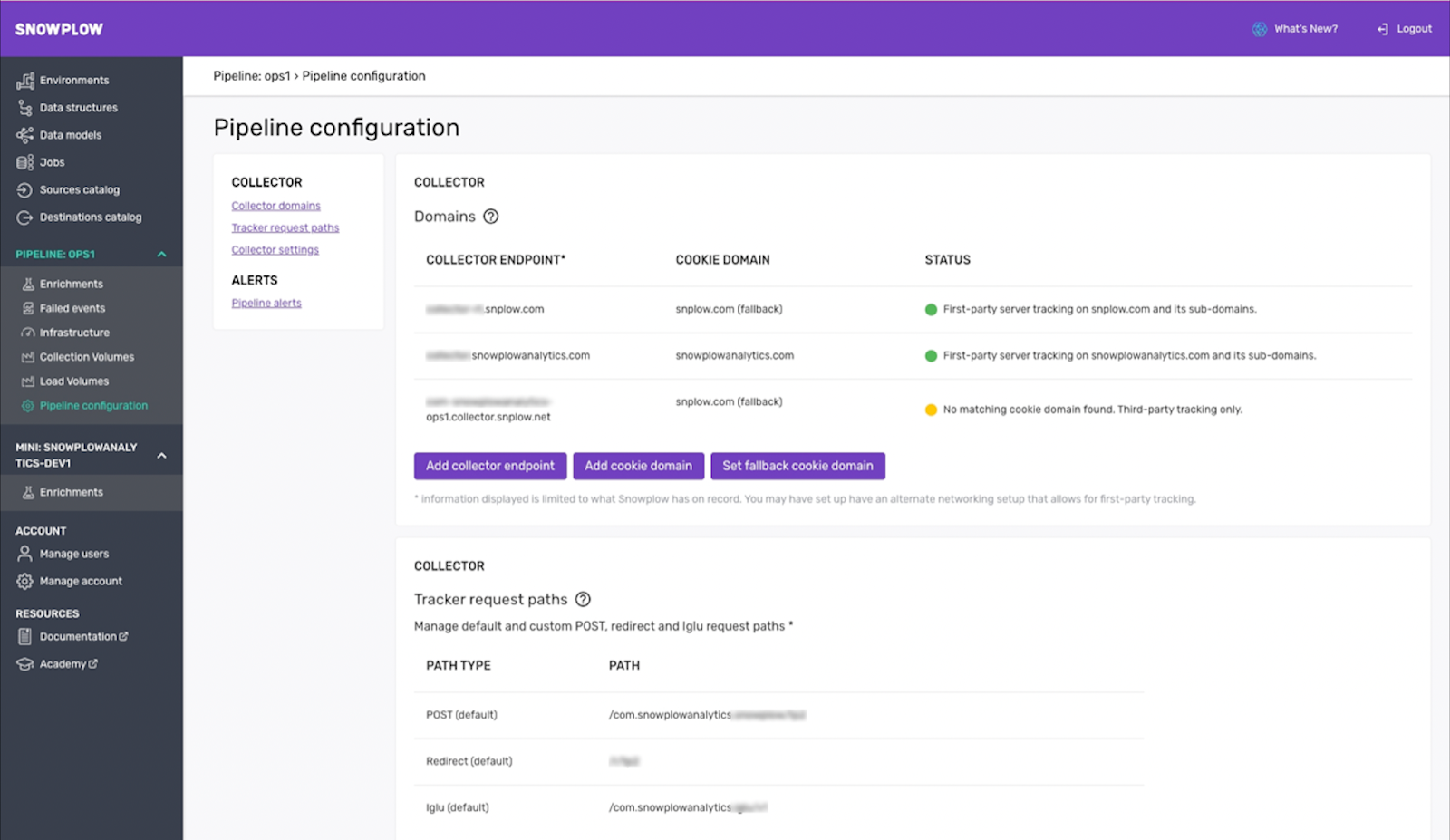
Snowplow is a behavioral data platform that allows you incredible control over how you collect and process real-time, rich behavioral data from different sources. This helps eliminate data silos and streamlines data organization so you can focus on what matters.
Features:
- Robust data validation and formatting features to ensure what you collect is accurate, meaningful, and can be acted on quickly.
- Complete visibility and control on your data pipeline, allowing you to easily evolve your infrastructure according to business needs.
- Collect rich events with 130 out-of-the-box properties, and add custom events tailored to your specific use cases.
Pros:
- You can define every aspect of your data management—from the structures to the modeling logic to the pipeline configurations. For General Data Protection Regulation (GDPR), you can even define rules around personally identifiable information (PII).
- Your real-time data can connect to warehouses and streaming services to power other tools immediately.
- It’s much more affordable than comparable software, whilst providing higher quality, richer data.
Cons:
- Given its breadth, snowplow requires buy-in from stakeholders across the organization to make it a success.
Business intelligence data management tools
BI (Business intelligence) tools allow you to make better strategic decisions by turning your key data into actionable business insights.
2. Tableau
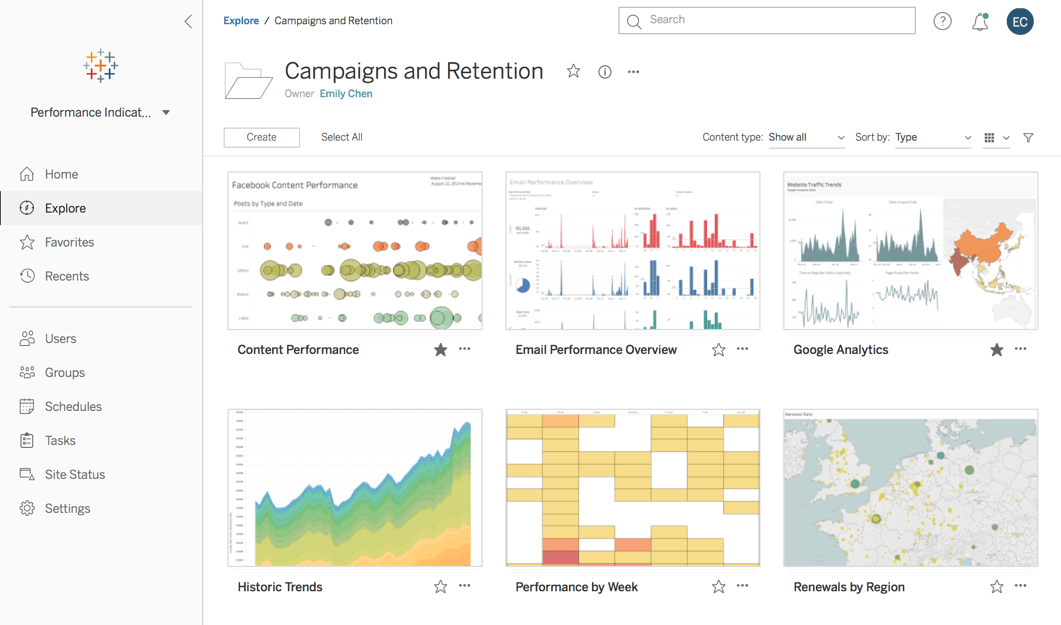
Tableau is a leading Business Intelligence (BI) platform that allows you to build shareable dashboards quickly and explore data from almost any system.
Features:
- Its natural language processing tool, Ask Data, allows you to ask for what you want without using any query language.
- Its forecasting tools allow you to quickly make predictions.
Pros:
- A strong support network—from training to its global community. There’s even Tableau Public, a visualization tool that’s free for anyone to use and learn from.
- An intuitive drag-and-drop user interface (UI) makes insights more accessible to any member of your team.
- Data visualizations are beautifully designed, helping you to display insights and shape decisions.
Cons:
- Although it’s possible to glean insights without much technical skills, ultimately, getting the most out of Tableau requires developer work.
- The more complex your needs are, the harder Tableau is to use—there’s a steep learning curve beyond the basic setup.
- Tableau might not be the best for organizations that are especially hands-on with their data structures and customization needs.
3. Looker
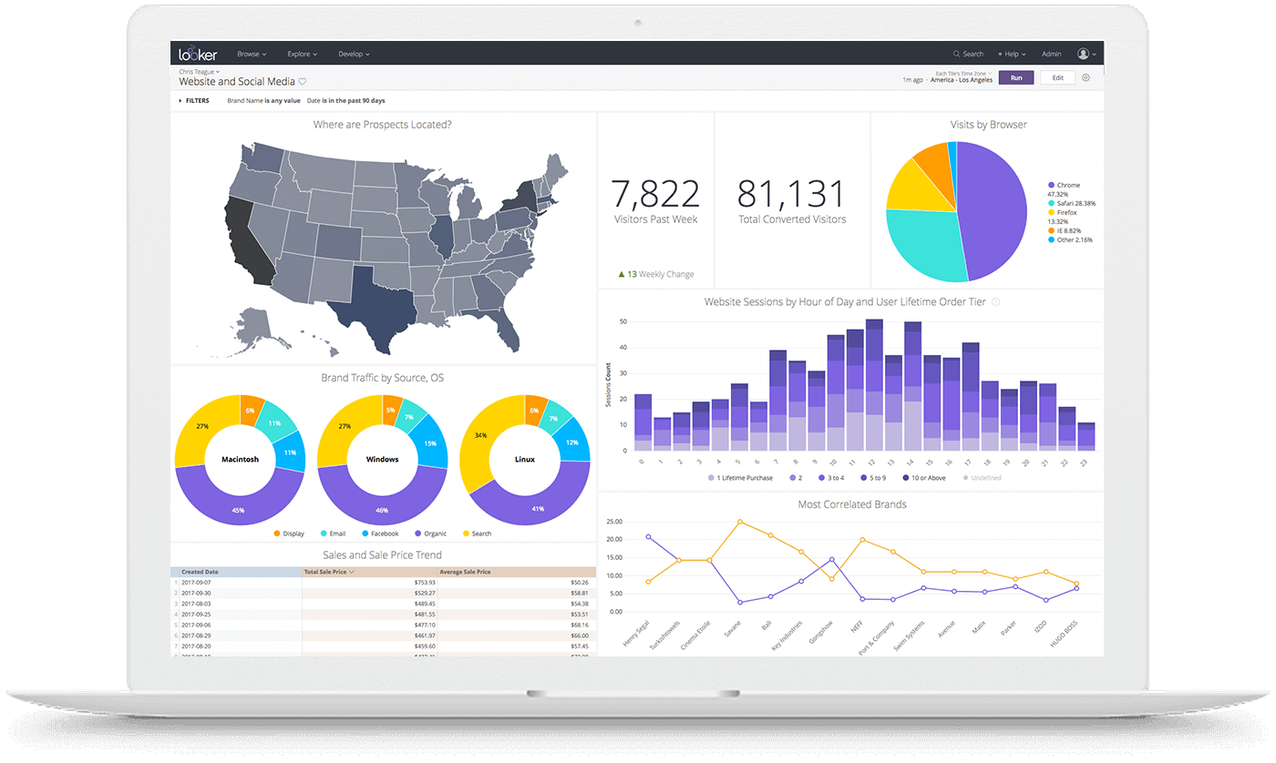
Looker is a BI platform that allows anyone on your team to explore data and build visually-engaging reports.
Features:
- Looker can create live connections directly to your database so you can query all of your data with ease.
- Dashboards are collaborative and shareable, with intuitive visualizations.
- JSON support and machine learning help you get more out of existing information with less finagling.
Pros:
- Extensive resources for business users to learn and get value immediately.
- Dashboards work well on multiple devices.
- Modeling language helps you better define the type of insights you want.
Cons:
- Can sometimes run slowly.
- Because it’s optimized for big data and complex queries, it can be expensive if your needs are simpler by comparison.
- Not as much training or support available compared to other competitors.
4. Power BI
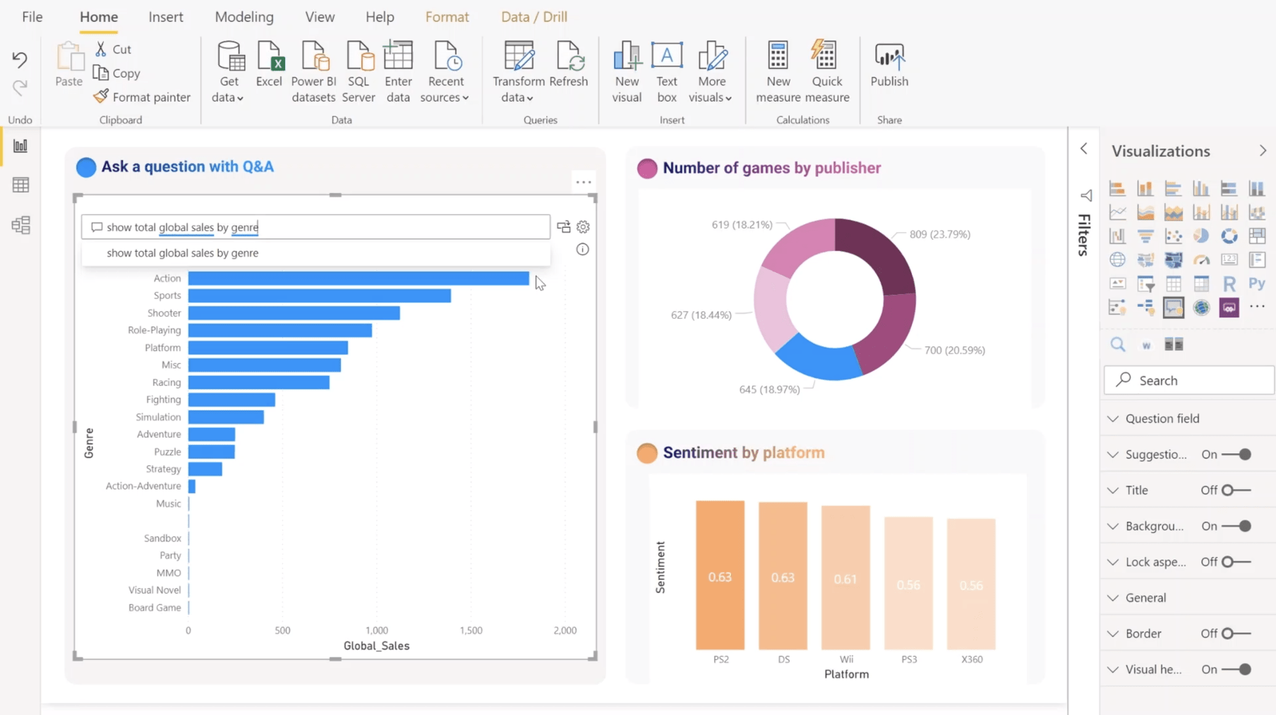
Power BI by Microsoft provides self-serve analytics for everyone from the individual to the enterprise-level team.
Features:
- Tight integrations and extensibility with Microsoft tools like Excel and Azure.
- Many security protections in place so you can control access at every level.
- Direct connections to many different warehouses and data files within.
Pros:
- Ability to completely customize your visualizations while also having easy-to-use drag-and-drop options.
- A good feedback loop from the strong community of users and frequent Microsoft updates.
- The Power BI embedded tool allows you to add reports onto sites, apps, and other lines of communication.
Cons:
- The UI is not as friendly or intuitive as competitors’ UIs.
- Limits when importing large data sets unless you upgrade to a higher tier.
- Steep learning tools on Power BI and also tools that help you get more out of it means high implementation cost.
Customer Data Platform
A Customer Data Platform (CDP) structures data across your entire organization, so you can better operationalize crucial processes, most often services and sales. Whereas behavioral data tools are optimized to model broad and real-time information for many business goals, CDPs are often set up to gather data into distinct user groups so you can target marketing efforts.
5. Segment
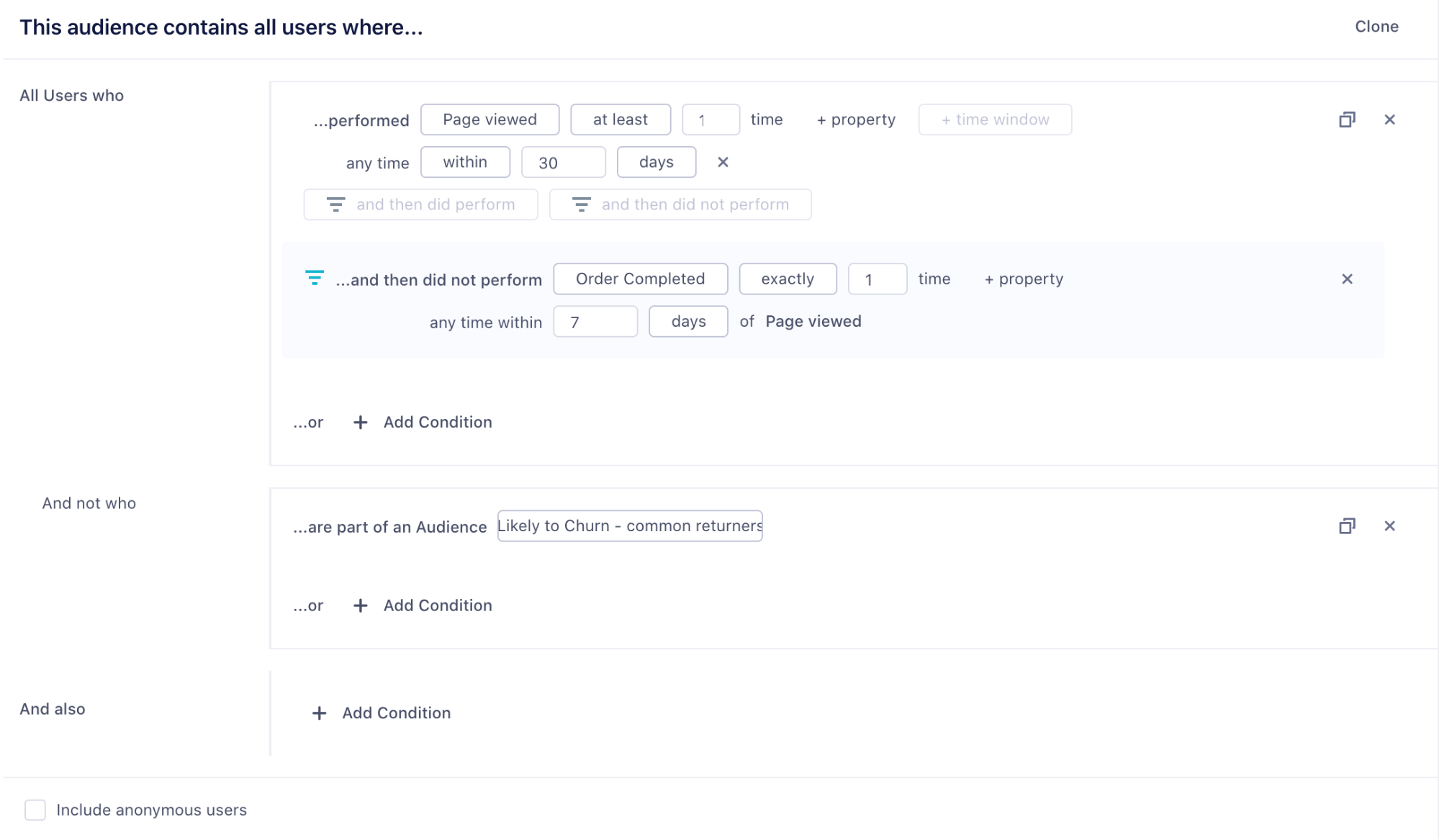
Segment is a Customer Data Platform that collects data about every customer touchpoint and allows you to create profiles, audiences, and more. These segments can then be deployed across all of your tools so that you have a complete picture of what customers are doing across disparate sources.
Features:
- Customer profiles allow you to easily see all of the activity, traits, and categories an individual belongs to.
- GDPR-compliant tracking means you can access and deploy information safely.
- You can quickly load data to a data warehouse with everything already properly validated from the rules you build yourself.
Pros:
- Easy installation so you can plug in and go.
- Friendly, intuitive UI.
- Plenty of native integrations to other popular platforms.
Cons:
- Some of the integrations aren’t fully optimized.
- You’ll need more technical expertise to customize or take full advantage of other features.
6. mParticle
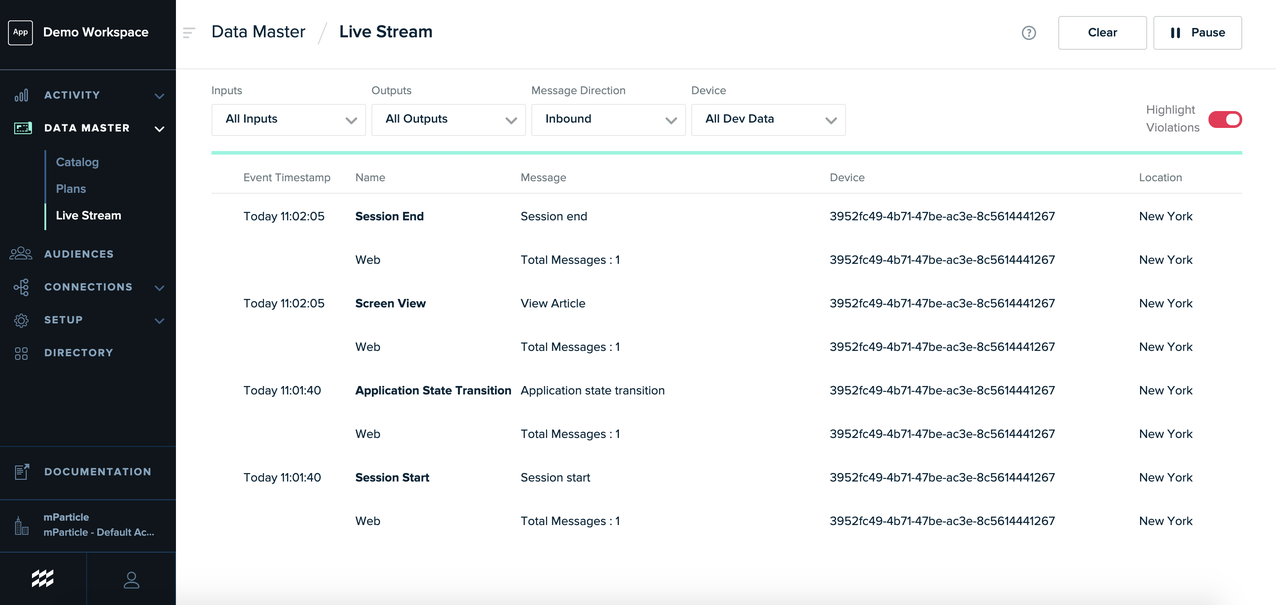
mParticle is a CDP that allows teams to provide customized experiences for their customers.
Features:
- Deep integrations with many apps mean you can connect more data streams.
- Built-in data transformation features.
- Multiple, helpful Application Programming Interfaces (APIs) such as user aliasing to help you get the most out of your data.
Pros:
- Greater control over refining your data and customizing it to your needs compared to similar tools.
- Easy filtering out of data you don’t want.
- High-quality, dedicated support helps you get started quickly.
Cons:
- Tons of features mean it’s difficult to know what’s usable.
- More transparency desired within in-app functions and error states.
Data warehouse management tools
A data warehouse is a central repository of information where the bulk of your data will be managed. Having all your volumes of data in one place is critical for analytics, reporting, and building tools.
7. Google Bigquery
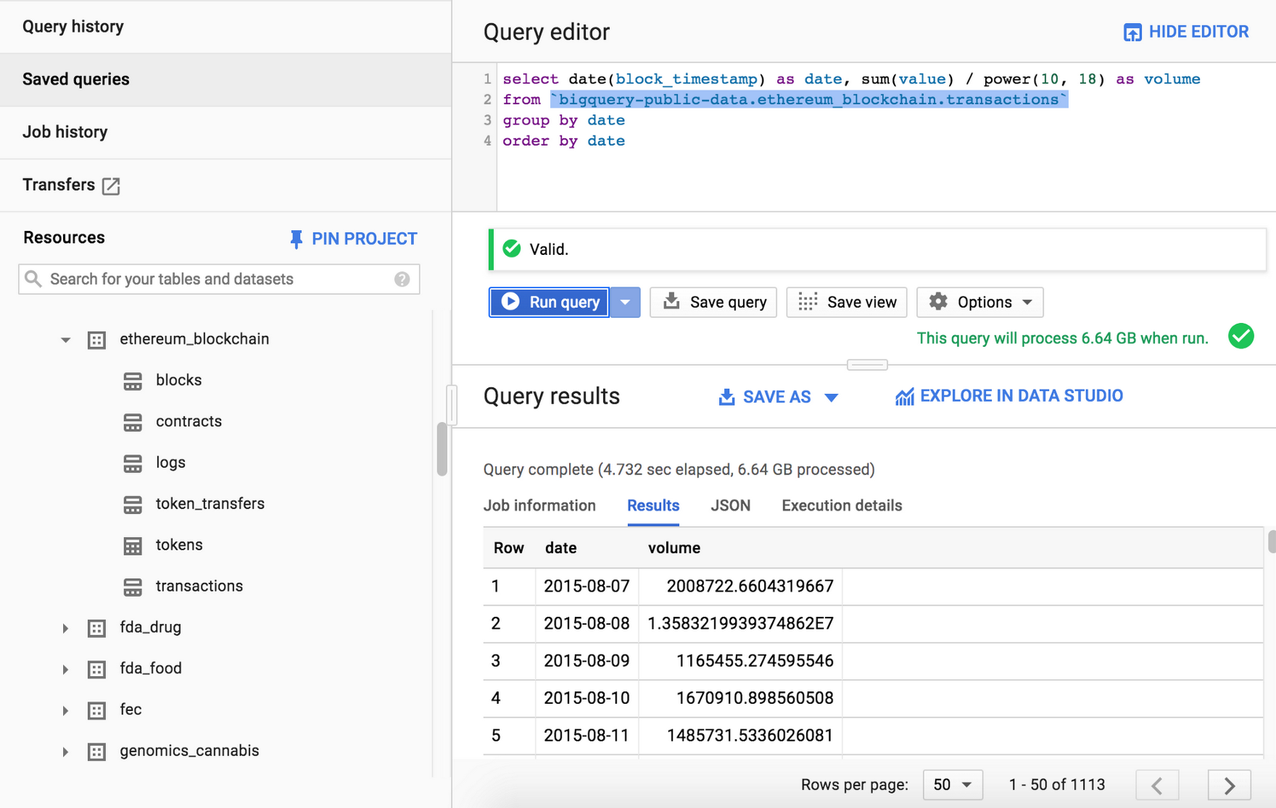
Google BigQuery is a multi-cloud data warehouse designed for businesses at scale.
Features:
- Fully managed relational databases in the cloud that you can query directly.
- A great choice for many companies that are already using other Google Cloud services such as Kubernetes.
- BigQuery ML provides predictions directly inside BigQuery.
Pros:
- With its connection to Google Sheets, anyone on your team can easily find insights without needing to know query languages.
- Robust and well-documented API.
- Relatively affordable compared to other big data competitors.
Cons:
- It can be difficult to integrate with other non-Google products, and the Google products that it does integrate with can sometimes be limited.
- Support can be tough to obtain, especially if you’re a smaller company.
8. Amazon Redshift
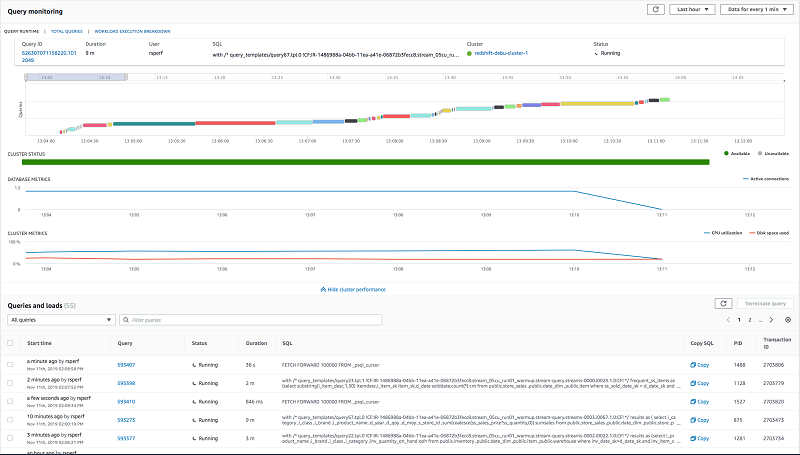
One of the most popular cloud data warehouses, Amazon Redshift offers high performance for businesses of any size.
Features:
- Flexible options with querying and writing data with strong workflows with S3.
- Federated queries allow you to search through data from many resources without needing to copy or write first.
- AQUA, the advanced query accelerator, allows for incredibly fast query response times.
Pros:
- Native integrations with Amazon Web Services (AWS’s) data tools make for seamless data pipelines and management.
- Speed in all areas is a bonus here—AWS will run everything both quickly and securely.
- Machine learning improves the speed even further by intelligently prioritizing queries to manage the load.
Cons:
- If you’re already using another data ecosystem like Microsoft or Oracle, Amazon Redshift might not make as much sense for you.
- Pricing doesn’t account for load fluctuations as finely or quickly as competitors.
9. Snowflake
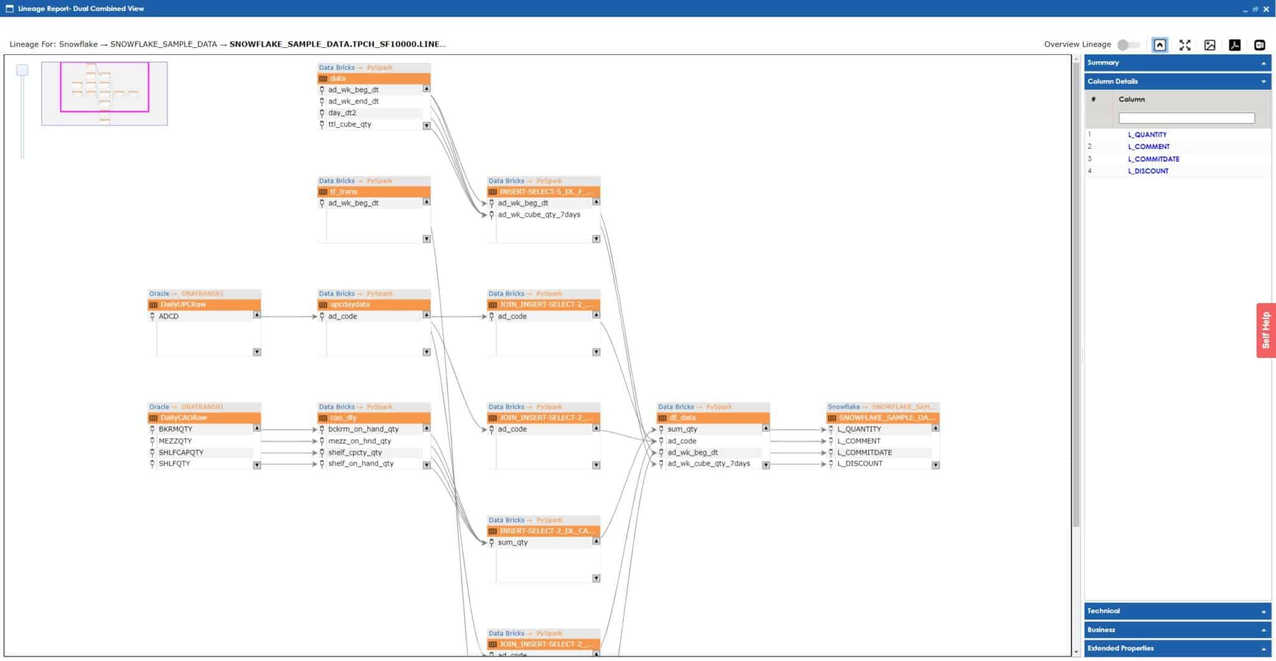
Snowflake is a popular data warehouse that breaks down silos between your data tools with multiple clouds.
Features:
- Increased control over processing queries and allocating necessary workloads with virtual warehouses.
- Comprehensive metadata for everything—from capturing changes to making cloning faster.
- Support for semi-structured data, like JSON, ORC, and Parquet.
Pros:
- Can handle large volumes of data easily because of the separation of storage and compute functions.
- Multiple cloud deployment options that also boasts high scalability.
- Very high-security compliance and fine control over access.
Cons:
- Native integrations could be improved, from function to getting popular ones on board.
- No options for macros or templates.
Product analytics data management tools
Product analytics tools allow you to get user and product insights straight from your data warehouse. It’s similar in some ways to business intelligence tools but allows you to have more complex queries on structures purpose-built for product concerns.
10. Indicative
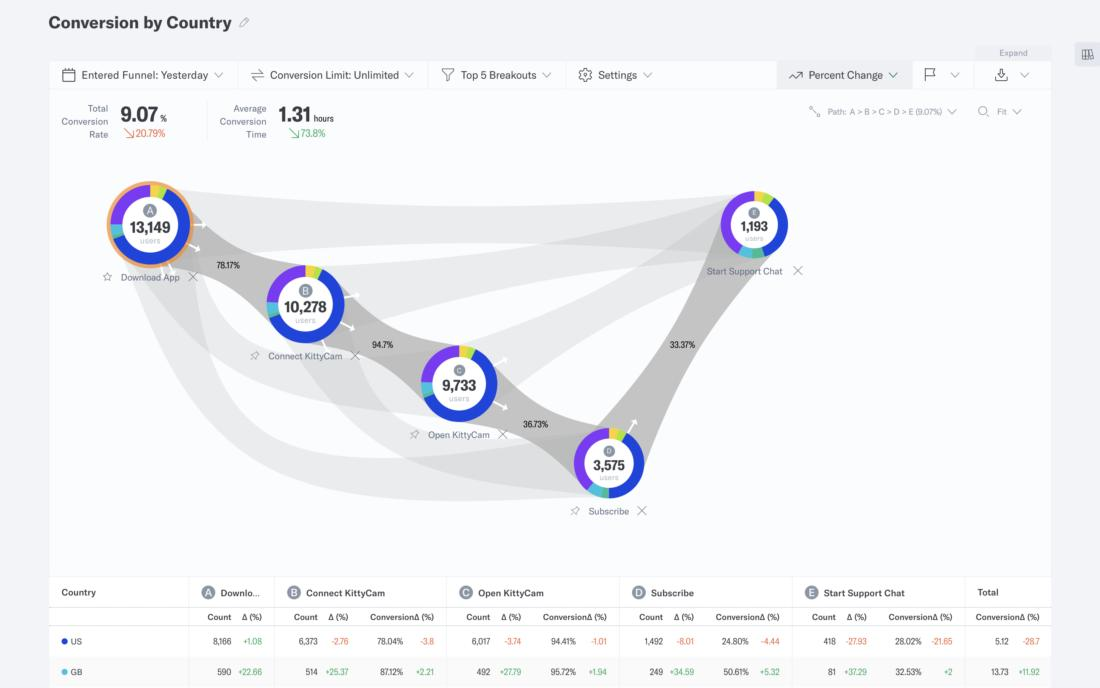
Indicative is a product analytics platform that allows you to view every part of the customer experience, from journey maps to cohorts.
Features:
- Collaborative, intuitive dashboards that allow you to drive insights from your metrics.
- Customer journey maps that are configurable without needing to know SQL.
- Segmentation and A/B testing reporting make for a powerful combo.
Pros:
- Every part of the tool is optimized to be usable for non-technical users, so you don’t have to wait on data teams to get your insights.
- Easily filter out data you don’t want, so everything stays relevant.
Cons:
- Onboarding can be confusing; although long-term use doesn’t require technical knowledge, you might need some starting out.
- Sometimes complex queries run slowly.
11. Rakam
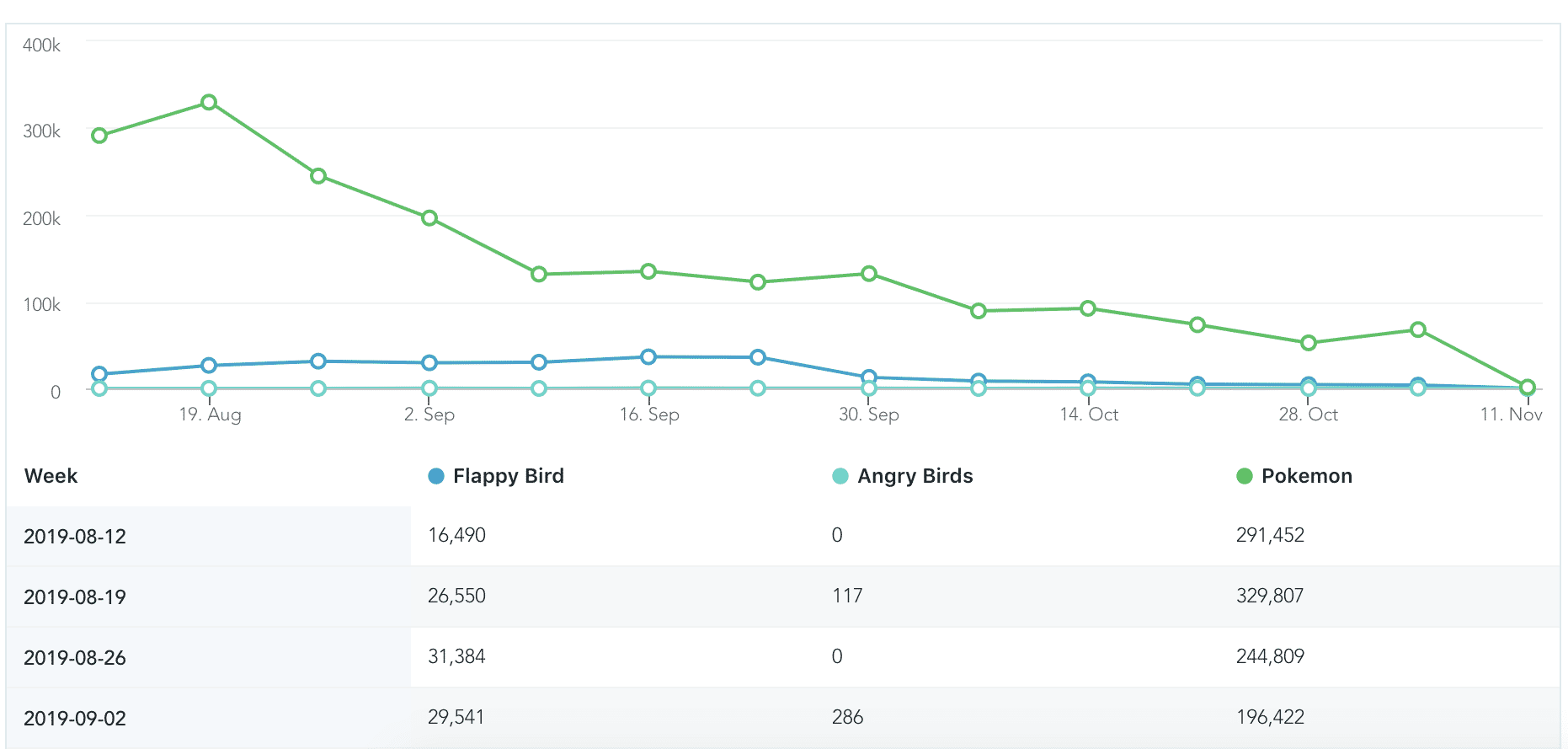
Rakam runs product analytics on your data sources that you can model into insights for your platform.
Features:
- You only need to model data once and don’t need to continuously run SQL queries.
- Analysts on your team can use visual tools to make charts, then export the generated SQL for later.
- Charts feature interactivity, so you can immediately take action from insights.
Pros:
- UIs and functionality are optimized to deliver value to people outside the data team, so they don’t have to depend on data resources.
- Plenty of integrations with other excellent data platforms such as Google Analytics and Snowplow.
Cons:
- Potentially slow customer service response times.
- As a newer offering, it doesn’t have the established community as the others in this category.
Data modelling
Data modelling is the process of aggregating event level data into structured, ‘modelled’ data which is simpler to query.
12. dbt
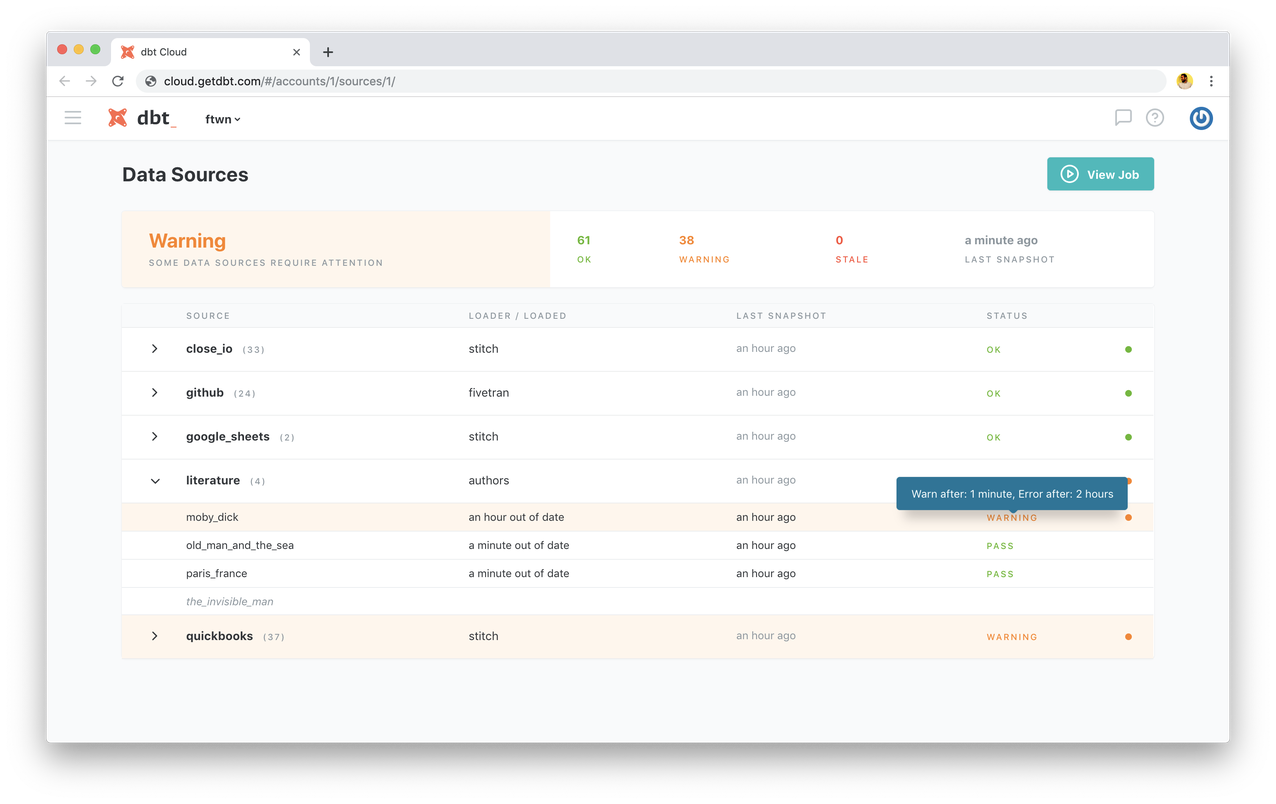
dbt is a tool that allows business analysts to easily transform and model their data using SQL. It tackles a very specific aspect of the data pipeline, so if you’re wondering if dbt’s the right tool for you, the team has a great blog post explaining precisely who they’ve built it for and why.
Features:
- Boasts speedy execution with modular SQL.
- The easy snapshots feature allows you to view historical data.
- Built-in alerts system so you can be notified of real-time changes.
Pros:
- Robust documentation on how to execute every area of the product.
- You can define macros for your organization to reduce the workload on frequent functions.
- Empowers analytics teams to model the data exactly how they need to for business contexts.
Cons:
- If you have especially complex transformation needs, dbt may be insufficient.
- It’s not built for streaming data, so there will be latency in results.
- It’s not enough if you’re looking for a complete ETL tool—for that, read on!
13. dataform
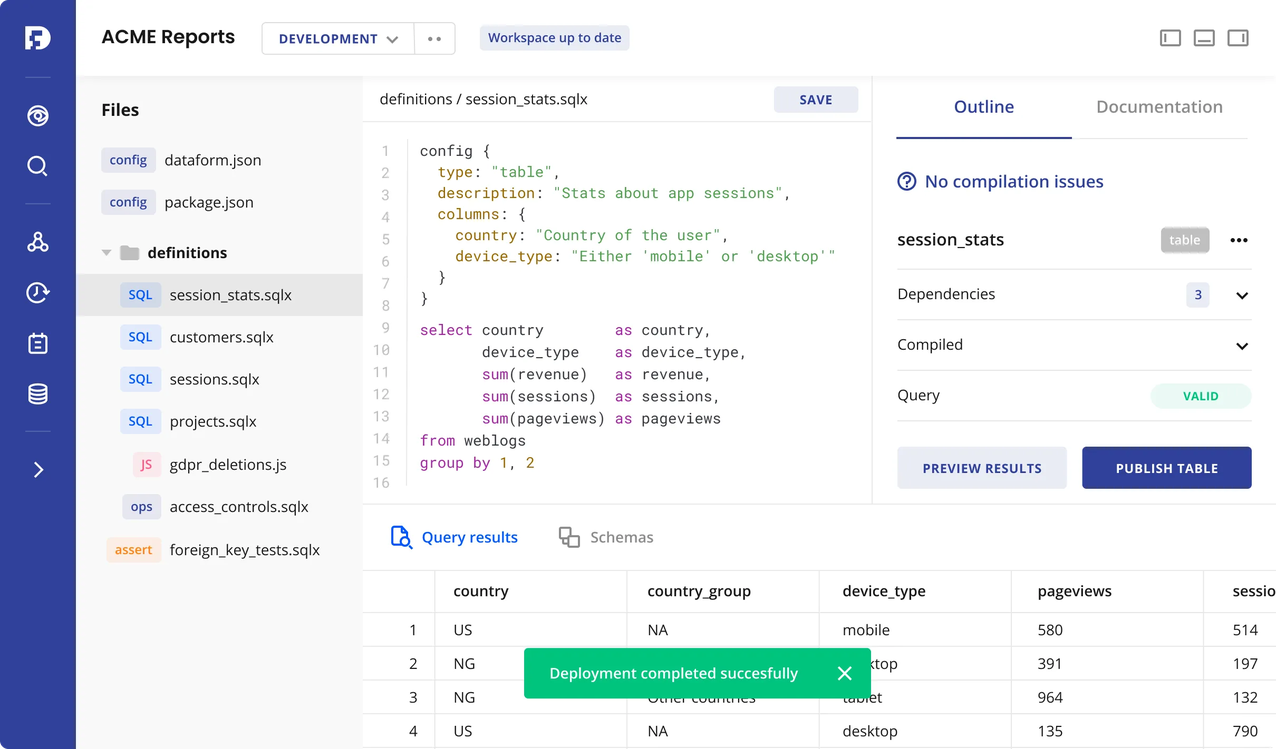
Dataform allows teams to build a single source of truth for all of their company’s data. It has recently joined Google Cloud, so it now bolsters an already impressive suite of data tools in the Google ecosystem.
Features:
- Dataform Web is a free option powered by open-source that can create centralized data models.
- Works with BigQuery to run functions like creating tables and testing.
- Extensible APIs allow you to customize Dataform to your specific needs.
Pros:
- The new partnership with Google Cloud means stronger BigQuery support going forward.
- You can set common SQL snippets to be reusable across the company.
Cons:
- The community of users is smaller and newer compared to others, so there aren’t as many online resources.
- Currently not available for self-host.
ETL and ELT
ETL stands for “extract, transform, load”—it’s the process by which you grab data from a source, make the format and models compatible with where it needs to go, and then stream it into the data warehouse destination.
14. Fivetran
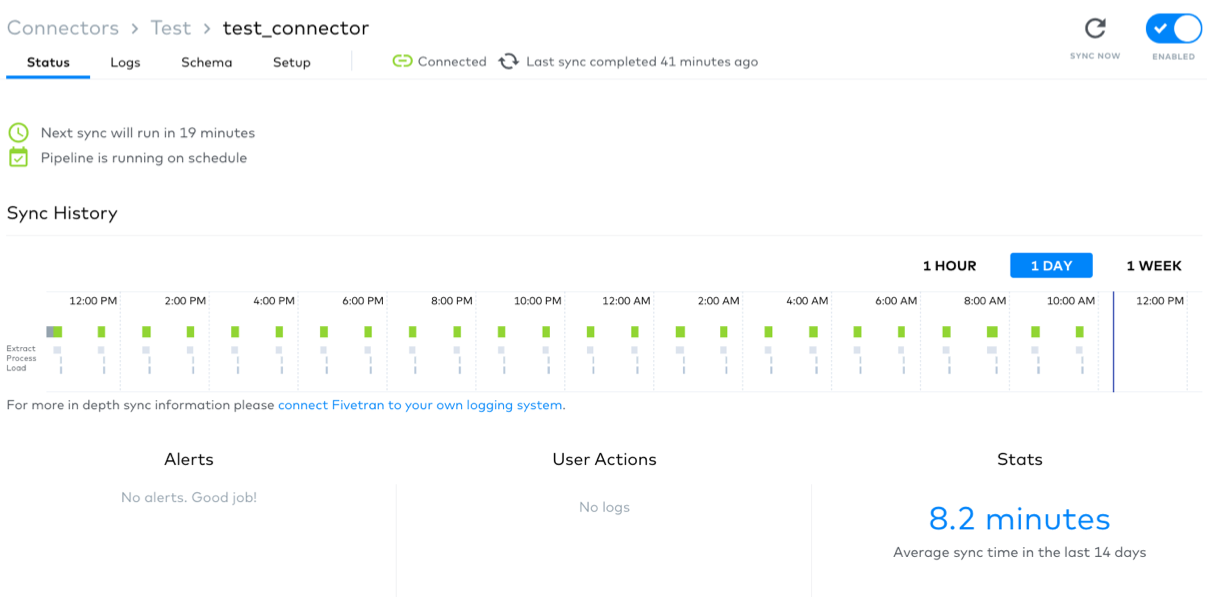
Fivetran is a leading ETL tool that helps teams unify their data systems at scale. With Fivetran, you can quickly connect and transform all your sources so you can start focusing on getting the best insights.
Features:
- Incremental batch updates mean speedier service.
- Schemas are both automatically normalized and migrated.
- Plenty of pre-built connectors.
Pros:
- Setup is both easy and quick compared to some competitors.
- Clear relationship diagrams make systems easier to manage and update.
- End-to-end encryption and enterprise security guarantees.
Cons:
- It may not be as well-suited to complex SQL transformation jobs.
- Works better with fewer data source systems.
- There isn’t two-way sync—for a tool that can go from warehouse to source, read on!
15. Stitch
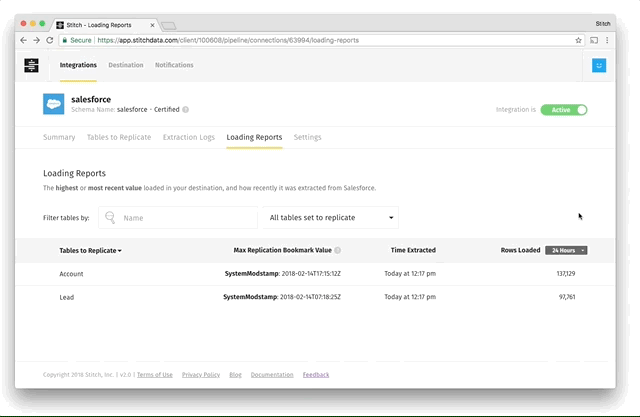
Stitch by Talend is a simple and extensible ETL that allows you to do more with the data you’re sending to warehouses with open-source connectors.
Features:
- Out-of-the-box integrations to commonly used tools are strong and sensible.
- Incremental replication reduces the load.
- Automatic scaling without having to manually adjust workloads.
Pros:
- Easy to set up on your own with no engineering assistance.
- Intuitive UI on tables makes them easier to manage, especially for teams that lack data team availability.
Cons:
- Might not work well with some info conversion or formula fields.
- Limited filtering options for data.
16. Airbyte
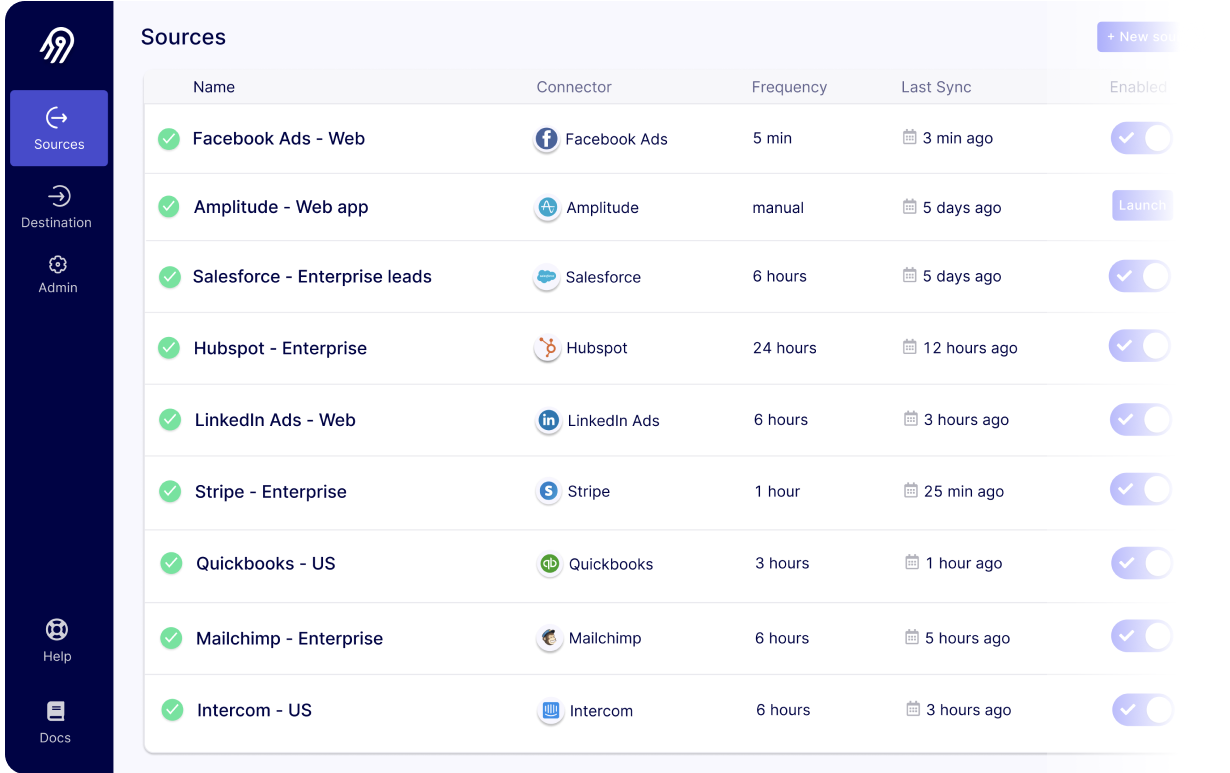
Airbyte is an open-source ETL tool that offers wide extensibility so you can customize the setup to your resources and needs.
Features:
- You can choose whether Airbyte normalizes your data or set up your own transformations.
- Many secure connection options, from native integrations to APIs.
- Scheduled updates and the option to manually re-sync gives you more control.
Pros:
- You can build custom connections using any language you want.
- It’s easier to immediately debug what’s happening in your data.
- Many integrations and a focus on developing these features even more.
Cons:
- Airbyte’s focus is more on the extract and load parts of ETL, less so with transformations. Some teams may need another tool.
Reverse ETL
A reverse ETL is just what it sounds like—instead of a tool that takes data from a source and makes it compatible for going into a warehouse, it copies data from the warehouse and writes it to a source. It gives operational nuance about what’s happening elsewhere when viewing information in a single source.
17. hightouch
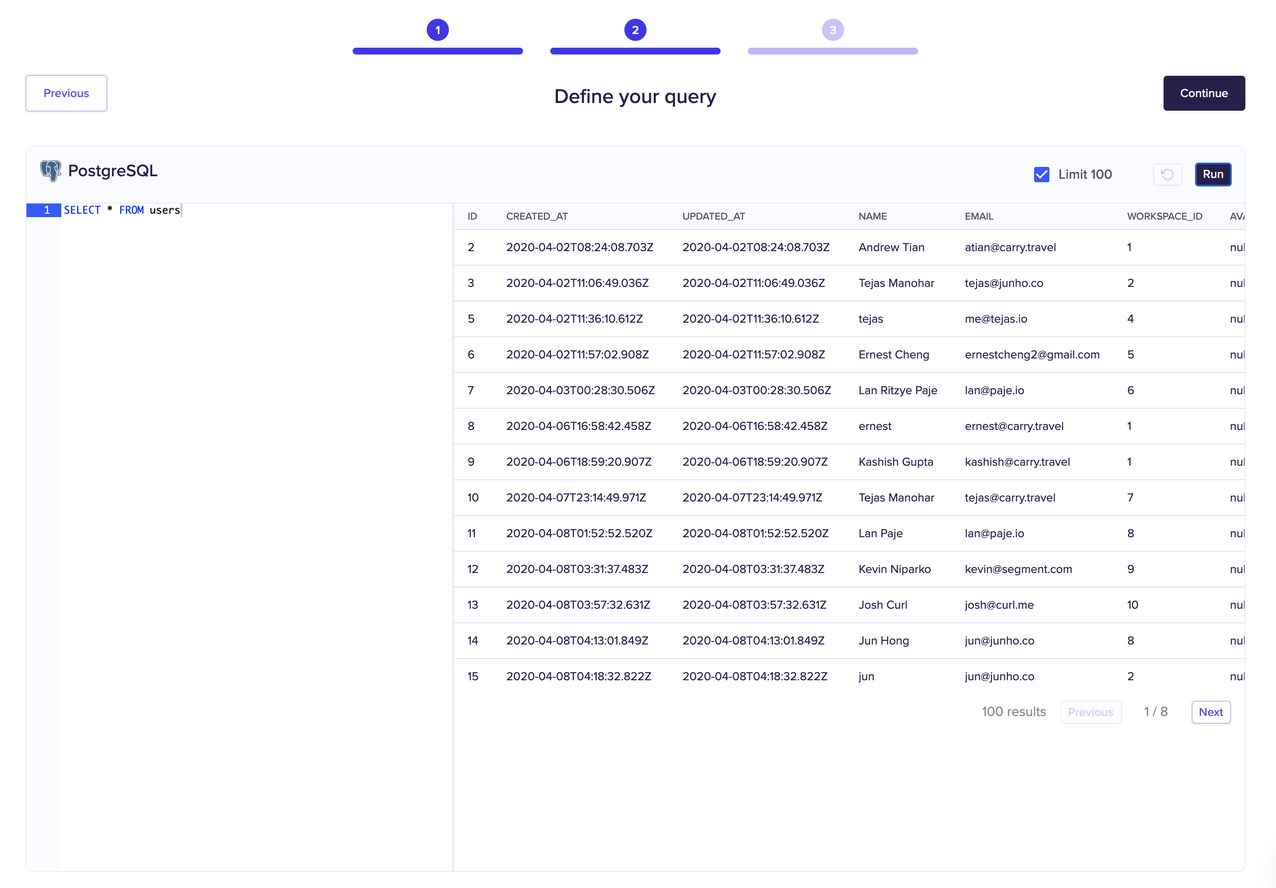
Hightouch is a reverse ETL that operationalizes your data so you can leverage better insights—all with SQL.
Features:
- Popular destinations supported, such as HubSpot and Salesforce.
- Two-way sync features allow you to manage directly in Git.
- Automatic error retries and rate-limiting means that issues don’t slow down the entire system.
Pros:
- A live debugger allows you to quickly identify changes and failures with more transparency.
- Native integrations with dbt, so you can use existing models in a dedicated system.
- All your queries are run directly in your warehouse for extra security.
Cons:
- It may not be sufficient for complex logic with transformations.
18. Census
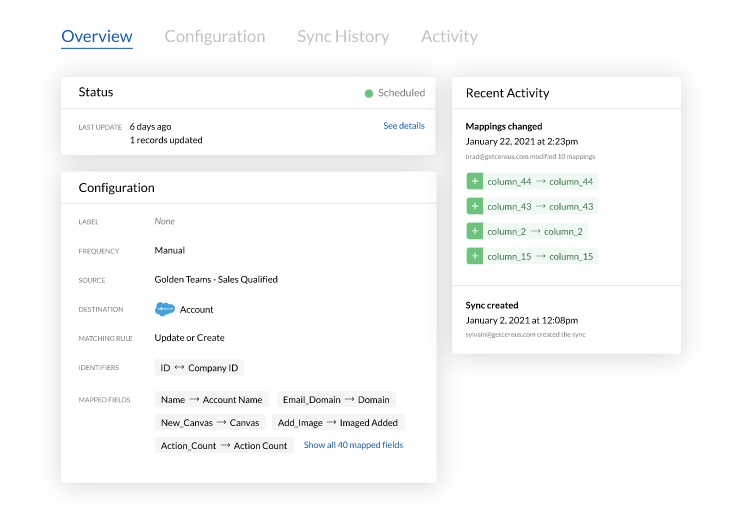
Census is an operational analytics platform that lets you send data from your warehouse straight into software as a service (SaaS) apps without needing help from engineering teams.
Features:
- You don’t have to wrangle all your apps’ APIs—all you need is SQL.
- 30+ native integrations, including a strong one with dbt.
- Incremental syncing keeps API usage low.
Pros:
- Although it's a young company, it already has an impressive roster of users, from Figma to Clearbit.
- Setup can be as easy as reusing the models you’ve already established with dbt.
- It offers a visual data mapper, so it’s easy to track what’s happening in your ecosystem.
Cons:
- It’s difficult to find online resources and community, as Census competes with results from national census operations.
- Census is a recently established company, so there may be some integrations that are still not fully built out.
Choosing the right data management tools for your stack depends on your needs
If your organization is serious about data, it needs to invest in the right data management tools. Although the tools you choose are ultimately dependent on your particular business needs, those listed above are highly recommended for most use cases. By aligning them as part of a modern data stack, you’ll enable better decision-making across your organization and pave the way for increased growth.