How Strava drives a culture of continuous improvement with self-serve behavioral data from Snowplow
Using Snowplow Behavioral Data Platform, Strava’s analysts can easily access and leverage massive volumes of rich, granular data.
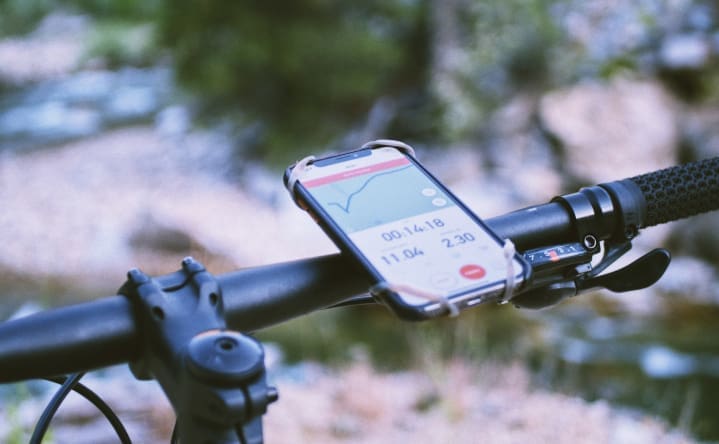
Background
Strava is the world’s most popular platform for athletes to record and share their sporting activity. Once dubbed ‘the social network for athletes’, today Strava is home to 64 million active users in over 195 countries.
Strava users record and upload over 40 million activities per week, making it a unique community for runners, cyclists and many other athletes to encourage each other, send a well-natured kudos, and compete among their friends.
Often we think a lot about operations, observability, all the things you need to make that thing work for your specific use case – but we didn’t always focus on being able to get the data to analysts, what the shape of those queries would be and what questions we would be asking of that data, and are we going to be able to provide the answers?”
DAVID WORTH | ENGINEERING MANAGER AT STRAVA
Challenge
For Strava, the world’s leading athletics app, collecting huge volumes of event data is a daily reality. A typical day will see 3 billion events (peaking to a high of 4.4bn) entering their data warehouse, fired from millions of athletes uploading their running and cycling activity.
In contrast, the team tasked with keeping this mountain of data accessible is relatively small. For Data Engineer Daniel Huang and Engineering Manager David Worth, it’s up to them to ‘democratize’ the data – making it available to analysts for reporting, dashboarding, and delivering insights for data consumers.
But the scale of this operation was a challenge in itself. Tables made up of hundreds of terabytes of data cannot be queried, and even if they could, they would be impossible to comprehend. And without robust infrastructure in place to handle those volumes, processes quickly fall apart and people cannot serve themselves efficiently. Data requests risk turning into long data breadlines of consumers waiting for support from engineers, which would not be sustainable without increasing headcount.
For Strava, engineering support for their analysts had to be kept to a minimum, but with their existing tooling, implementing tracking on new features proved tricky and unintuitive. This difficulty led to ‘analytics blind-spots’ where pieces of the analytical puzzle were missing. For an organization like Strava, where data is
critical to a culture of continuous optimization, the ability to implement tracking easily, without missing key features, was crucial.
Solution
Strava’s requirements, namely to manage data collection at great scale, without incurring huge costs, eventually led them to Snowplow. Snowplow’s technology in many ways is the opposite to Strava’s previous vendor. Where their previous solution was a black box, Snowplow is open source and flexible. Where the previous costs scaled as volumes increased, Snowplow offered fixed, tiered pricing.
Most importantly, Strava’s journey to democratizing data runs more smoothly with Snowplow. Once they became familiar with defining custom events and entities, data analysts were quickly able to instrument end-to-end tracking by themselves, without any help from the data engineering team. Now they can take slices of the data they need from the central platform, (thanks to derived tables in Snowflake built by David’s team) and break the data into manageable ‘chunks’. This setup gives Strava analysts the autonomy and freedom to serve data consumers efficiently, which is vital to the business.
Not only does this free David and his team from endless enquiries, but it means analysts are able to keep pace with the constant evolution of new features that is part of everyday life at Strava.
Setting up tracking with Snowplow has proven to be far easier and far less painful than previous solutions, which means new features or product iterations don’t go missing under the analytics radar.
We would not have achieved our current level of self-serve data without Snowplow. It has enabled us to democratize our data culture, significantly improving our analytics coverage and deepening our insights.”
DANIEL HUANG | DATA ENGINEER AT STRAVA
Why Snowplow?
While Strava’s data team has the expertise to run Snowplow on their own, for Engineering Manager David, having Snowplow’s managed service was much more cost effective than hiring a full-time engineer (or multiple) to take care of the pipeline. More importantly, it also means that Strava’s small data engineering team can focus their resources on meaningful projects that move the dial for Strava’s analytics, rather than managing infrastructure.
The Snowplow future: real-time data, powering more use cases
The self-serve data story at Strava is going well, but for the data engineering team, the journey is far from over. Their next ambition is to use Snowplow to deliver real-time data to power use cases for the machine learning and product teams. Leveraging real-time data will allow their machine learning team to benefit from instant feedback on certain features, and perhaps enable other use cases such as anomaly detection alerts, which Daniel has already explored for key metrics.
As for the sky-high data volumes, with some days peaking at around 4.4bn, Strava is closely monitoring the number of events coming through, and may limit or even reduce the number of raw events they’re collecting. But for now, Snowplow’s infrastructure enables the team to manage a large-scale, enterprise data platform that helps keep Strava at the top of the industry.
How you can get started with Snowplow
To learn more about how Snowplow can empower your organization with behavioral data creation, book in a chat with our team today. Alternatively, Try Snowplow is our free, easy-to-use version of our technology, which allows you to create your own behavioral data in under 30 mins.
Customer Case Studies
Explore real-life success stories from companies using Snowplow.