The challenges around understanding user behavior on mobile
In the previous chapter, we looked at the importance of mobile as a platform for companies to engage with their customers. We also reviewed the existing tooling available to data teams to deeply understand how users are engaging with mobile applications to create more meaningful and engaging experiences. In this chapter, we will dive deeper into some of the challenges encountered in mobile analytics today.
Understanding the customer journey
Reliable user identification
To date, user identification on mobile relies primarily on various device identifiers. On iOS, Apple provides the IDFA, a user identifier for advertisers that unequivocally identifies the device across apps, and the IDFV, an identifier for vendors that identifies a user across apps from the same vendor only. On Android, there exists only the AAID (or GAID on Google devices) which is similar in functionality to IDFA but can be reset by the user in the settings.
However, this reliance on platform-provided identifiers may no longer be a viable strategy for identifying users. Apple’s new App Tracking Transparency policy (introduced in April 2021 in iOS 14.5) requires apps to ask users for permission before tracking user behaviour for the purpose of sharing it with third parties, including tracking the IDFA. Initial data suggests that only around 10% of users globally are happy to accept such tracking when prompted. And this is only the latest user privacy related restriction Apple is placing on the App store. Back in 2019, Apple restricted the use of ads and third party trackers in iOS apps aimed primarily at children. This poses a huge challenge to existing third-party analytics providers, including the marketing and product analytics tools discussed in the previous chapter. As user privacy becomes more of a focus for large tech organizations, companies need to re-evaluate how they can set out reliable mobile analytics and consider switching to first-party solutions.
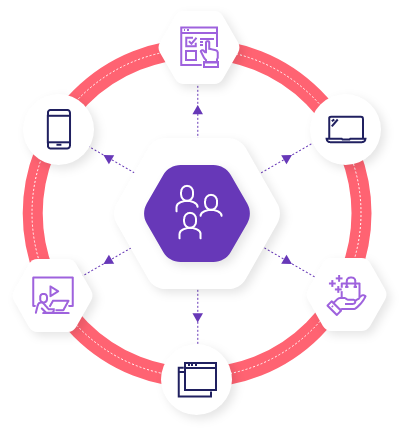
Attributing in-app activity to marketing touchpoints
One of the key use cases for identifying users is the ability to measure the effectiveness of marketing activities. Marketing attribution is already more complex on mobile than on web as typically there is a step between the ads users see (on social media, in the mobile browser or within other apps) and the conversion – the app install via the app store. Existing marketing solutions stitch user activity together using deferred deep links. However, these links rely on the identifiers for advertisers to stitch the user journey together. Specifically, to link the metadata captured by the attribution provider before the user is sent to the app store with the data collected from within the app subsequently. As these identifiers lose their reliability, companies need to resort to the measurement options the platforms themselves provide, such as Apple’s SKAdNetwork API, and move away from user-level attribution to focusing more on cohorts and campaigns.
Bringing user activity in-app into context
Another key use case for identifying users is linking their in-app activity to their wider customer journey to provide more engaging and valuable experiences for users. For example, companies may want to link in-app behaviour to activity on web, interactions with email or engagement with Customer Service. Even if these activities all occur within first party systems, and therefore users can be identified reliably using internal user identifiers, challenges remain. Typically most companies will be using different tools to track different aspects of their customer journey. This creates data silos: data sets from different platforms are stored in different locations, at different levels of granularity, in different formats, etc. This makes it challenging for data teams to look at the customer journey in its entirety, and align strategies across Marketing, Product and Customer teams.
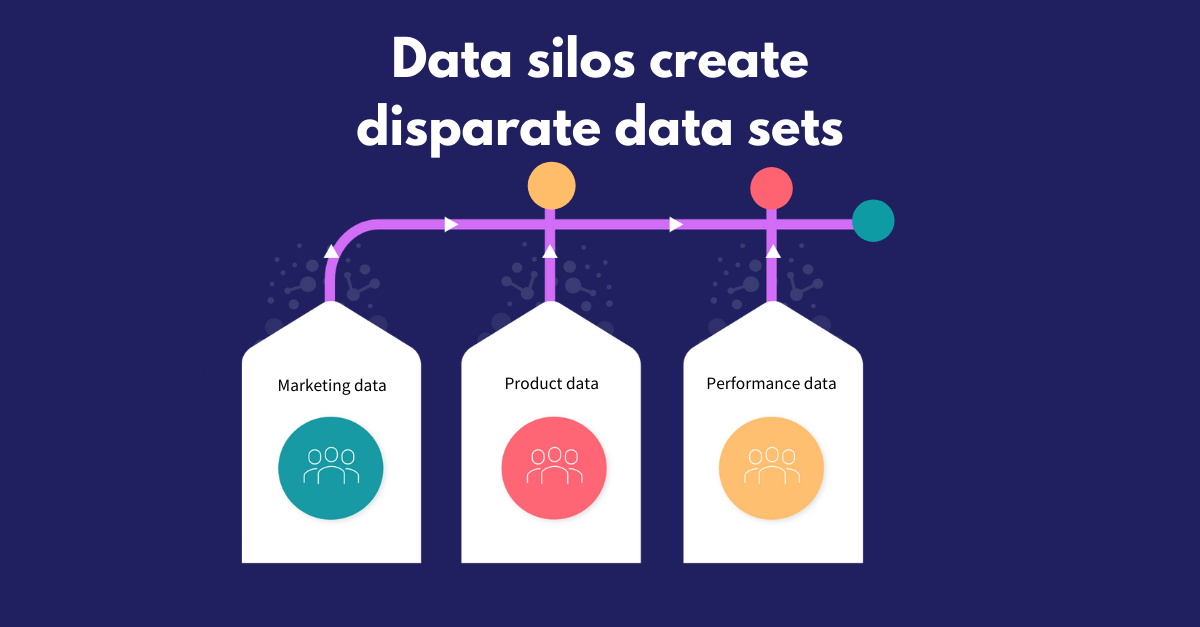
Dependency on developer resource
Another challenge around understanding user behaviour on mobile is the reliance on mobile developer resources in deploying trackers on mobile applications. Unlike on web, there are no tag management solutions optimized for mobile applications. Furthermore, at least on iOS, apps need to go through the app release cycle, which means tracking updates cannot be made whenever the developer wants, and any data quality issues require a new app release – forcing users to upgrade their apps to resolve. Thus, thorough testing and QA are necessary, and many analytics tools do not have adequate support for such processes. All of these challenges make it difficult for companies to reliably collect user behavioural data from their mobile applications, and to stay agile with the information they capture as the apps themselves as well as their understanding of them and their analytical requirements evolve.
Mobile analytics is not web analytics on mobile
Most analytics tools started on web, and so they naturally have web-centric concepts and assumptions deeply embedded. Specifically, they often assume different user behaviour and focus on different core metrics. For example, measuring sessions and conversions rather than customer lifetime value and retention. Furthermore, they often don’t offer sufficient customization capabilities. Mobile apps display much greater variation in design and functionality than websites.
For example, a mobile banking application such as N26, an exercise tracker such as Strava and a mobile game such as Peak all look and feel completely different. The amount of time users spend in these apps is different, how they interact with the apps is different and what highly engaging experiences in these apps look like is completely unique to the app itself. Therefore, collecting data in a generic format predefined by a given analytics provider will not be suitable for capturing granular interactions that make up user behavior in these rich, unique mobile experiences.
Coming next
Together, these obstacles make reliable mobile analytics significantly challenging for modern businesses to navigate today, especially if relying on packaged analytics tools. In our next chapter, we'll explore the opportunities in building a robust behavioral data asset, and how organizations can overcome barriers to effective mobile analytics.