5 advantages of Commercial Open Source Software (COSS) over SaaS for data analytics
What is Commercial Open Source Software (COSS)?
The Modern Data Stack is evolving at a pace that is hard to comprehend. Every day, new companies pop into existence, serving a novel niche within complex data pipelines.
The overwhelming majority of these tools are considered Software as a Service “SaaS”, and their codebases are proprietary – other companies pay a subscription to use the products. With greater moves to customization, compliance, and data security, however, many more companies are moving to software providers with an open-source core. This essentially means that the main codebase is open source, with additional functionality, services, and support provided over the top of this for a fee.
COSS is like the third way between open source and SaaS. It avoids many of the drawbacks of both types of solution, while also borrowing from the benefits. The products themselves are virtually indistinguishable from SaaS products in many cases, the differences come from factors like the open-source community and the transparency of having open-source code at the heart of the tool.
Open-source software has grown in credibility, with some of the big names in data using the COSS model to monetize their products while simultaneously making them available to the whole world for free (see the list below).
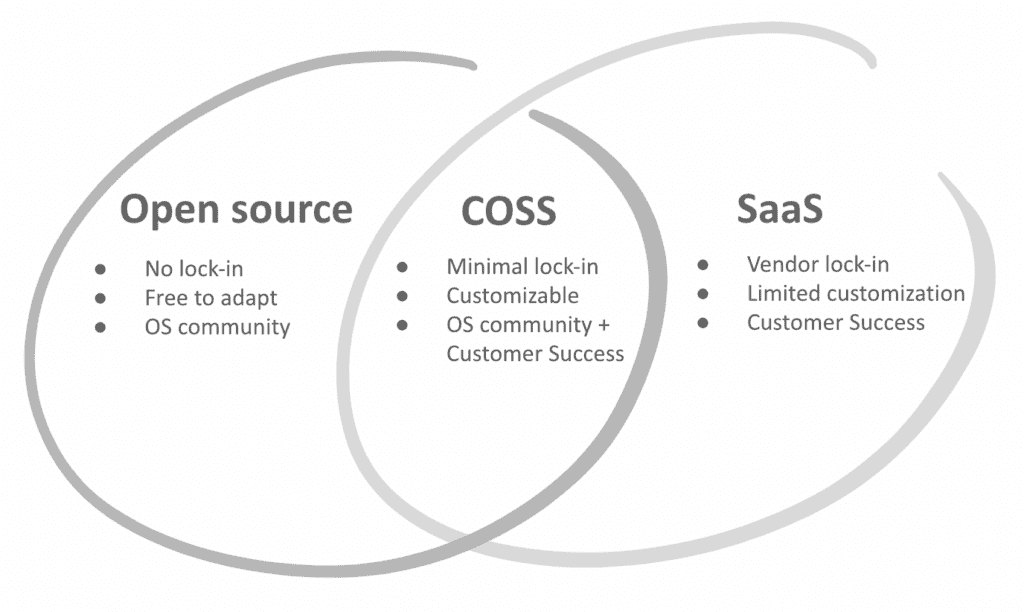
Advantages of Commercial Open Source Software
1. A range of deployment options
There are massive advantages to being able to inspect the codebase of a product before you deploy software. After this, you can even run the software open source as a proof of concept to see whether you would get enough value from the open source alone. If the paid services or functionality are felt to be lacking after the trial, then upgrading to the paid product can be done with the knowledge that the software is useful for the company.
2. Flexibility and customization
Open-source software has a huge degree of flexibility. Organizations can adapt and modify software to meet their specific needs. Unlike purely commercial SaaS solutions that may enforce rigid definitions or workflows, commercial open-source software empowers companies to tailor the software according to their unique requirements. This flexibility ensures that the software aligns seamlessly with existing processes, and ultimately optimizes for efficiency and productivity.
3. No Vendor lock-In
Long-term technical debt is easy to accrue and very hard to shake off in the world of data. Many companies end up using tools that actually hold them back for fear of ripping off the bandage. COSS companies generally do not have any vendor lock-in and it’s therefore easy to move on, should you need to.
4. Wide adoption and community
Open source generally means wide adoption, and this allows COSS companies to learn from their large user bases. The benefits of this are huge, as you generally know you’ll be using a battle-hardened product that won’t fail when it comes to a weird use case the Vendor hasn’t thought of. The wider community will often contribute support, ideas, and plugins. Furthermore, the communities behind OS products often contribute to the codebase, further strengthening the whole product.
5. Security and auditability
The transparency inherent in COSS tools also creates greater security. Security experts and auditors can choose to review and analyze the code, identify vulnerabilities, and suggest improvements at any time. The scrutiny of the collective, in this sense, reduces the probability of backdoors or malicious code being present. The community can also contribute to greater security as there are more eyes on the code. Finally, due to the level of customization possible, companies can often configure the software to their own security needs (this is certainly the case with Snowplow BDP).
Examples of Commercial Open Source data companies
1. Snowplow
A tool to create first-party customer data in real time to power next-gen digital analytics and customer engagement. Every action a prospect or customer takes on a digital interface (web/apps/IoT, etc) is captured along with over 100 contextual fields. This can be used to create advanced data products and ML models.
2. MongoDB
A document-oriented NoSQL database platform for storing and managing large volumes of structured and unstructured data.
3. NoSQL database platform
Like MongoDB, another scalable solution for storing and handling structured and unstructured data at scale.
4. Elastic
Elastic has an open-source search and analytics engine called Elasticsearch allowing companies to perform real-time search, data analysis, and visualization on large datasets. They also offer tools such as Kibana and Logstash for log analysis and data processing.
5. Databricks
A unified analytics platform based on Apache Spark – an open-source big-data processing framework. Their platform enables data scientists and analysts to perform large-scale data processing, machine learning, and collaborative data exploration.
6. Confluent
The company behind Apache Kafka, an open-source distributed streaming platform. Widely used for building real-time data pipelines and streaming applications.
7. InfluxData
The company that created InfluxDB, an open-source time series database. It is designed for handling high volumes of timestamped data, making it suitable for use cases such as monitoring, IoT, and real-time analytics.
8. Grafana Labs
Grafana is an open-source data visualization and monitoring platform allowing you to create interactive dashboards and visualizations for analyzing and monitoring data from various sources.
Why Snowplow chooses to adopt the COSS model
Snowplow has always been built on an open-source core. This led to our tracker being the third-most used in the world. This widespread adoption has supercharged the growth of Snowplow BDP – our paid product – and meant our users can collect the most accurate and detailed customer data on the market.
As part of this, our 10,000+ users contribute to a vibrant data community and are pushing forward the art of the possible with behavioral data.
You can now try Snowplow completely free to see how our ultra-granular data looks in practice (it takes just 30 minutes to set up).