Simply put, companies that adopt warehouse-first advanced analytics for their websites have a level of insight not open to those relying on simpler, packaged solutions.
The adoption of warehouse-first analytics is rapidly gaining traction among businesses of all sizes, as organizations strive to break down data silos and gain a holistic view of their customers and operations. According to a recent survey by Forrester Research, 65% of businesses are planning to adopt a warehouse-first approach to analytics within the next two years. This shift is driven by the increasing volume and complexity of data, as well as the need for more sophisticated analytics tools to make sense of it all.
The challenges with advanced web analytics
In order to undertake advanced web analytics, companies need to create an accurate representation of how users move through online experiences. These efforts can be limited by various challenges, such as privacy, tooling limitations, and the complexity of user journeys.
1. Browser restrictions
Changes such as ITP, browser cookie updates, and privacy software all lead to complications when making business decisions based on web analytics. With ITP, for example, cookies can be restricted to less than 7 days for Safari users, as well as those using other privacy-conscious browsers. This skews your data for what can often be your highest-value customers, often inflating figures and misleading your attribution efforts.
2. SaaS apps often use black-box logic which you don’t control
Many packaged web analytics tools are black boxes, meaning that organizations have no idea how their data is being used and transformed, or what assumptions are being made. Not being able to control how your data is defined can seriously limit your analysis. Take the length of a session, for example, or whether an event fires on click or page load – all these things can make a difference to companies serious about advanced analytics.
3. The complexity of user journeys
Cross-channel and multi-devive analytics pose a further challenge. When this is done incorrectly, it can cause data siloes across a variety of tools and storage destinations. This means effective analysis of your data is not possible and that you have to ask more limited questions of your data sets.
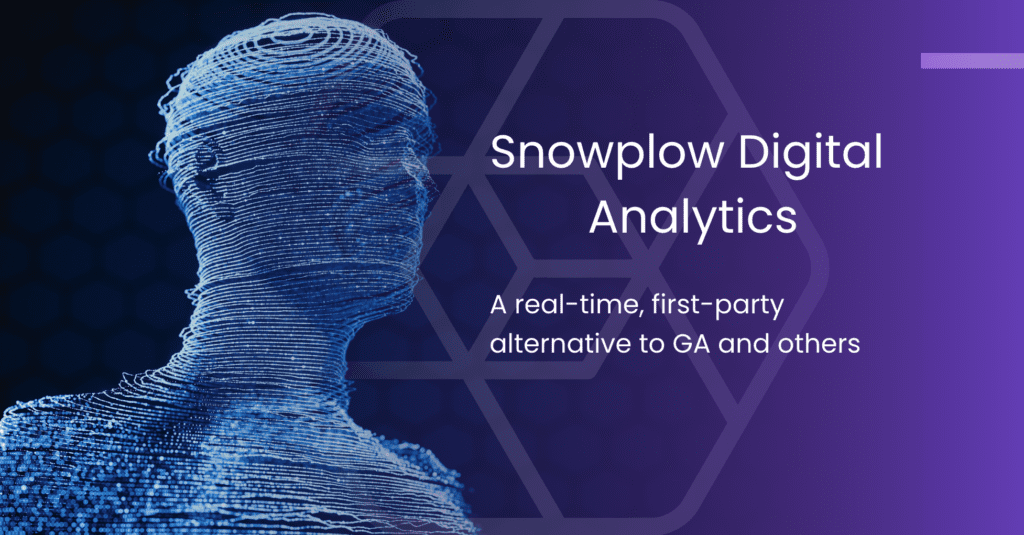
Snowplow Digital Analytics
Snowplow has traditionally been a favored tool of data teams, but our latest solution, Snowplow Digital Analytics, is made for marketers.
All the dashboards and metrics you built your GA reporting on are available in Snowplow. These are real-time, more compliant, and fully warehouse-first. You can build on top of these GA-style dashboards with full flexibility as you evolve your advanced analytics and AI use case
The opportunity of creating warehouse-first web analytics
Relying completely on a single SaaS application for your web analytics locks you into a tool that is not best-in-class for each function it provides, and may pose issues with black-box logic, as discussed above.
The warehouse is becoming the center of data gravity, with the global data warehousing market set to be worth $30 billion by 2025 (Global Market Insights).
When companies adopt a warehouse-first approach to behavioral event data, the structure of the pipeline might look something like this:
After the data warehouse, the data can be integrated with 3rd-party tools, such as your Martech stack.
With this warehouse-first approach, you can use the best tool for Data Creation, and data storage, as well as for integration with other tools (such as reverse ETL).
This can empower every department to answer more nuanced questions, as data analysts can slice and dice the data with SQL, Python and R in your storage location(s). This provides a level of granularity simply not possible with traditional packaged analytics solutions – such as Google Analytics.
Another key advantage of being warehouse-first is compliance. With a modular pipeline, you can choose the most compliant tool based on your context and store your data in the appropriate jurisdiction – for example, anyone capturing data on EU citizens generally needs to store this data in the EU.
What is a Data Product Accelerator for web analytics?
A data product is an actionable data set you can create through advanced user tracking.
It is the foundation of a data application, which could be a churn- prediction model or marketing-attribution model, for example.
A Data Product Accelerator, or DPA, is a guided recipe that helps data teams to undertake advanced analytics and set up a customizable dashboard by following a simple, step-by-step process.
Ultimately, you can build a deeper understanding of customer behavior on your mobile apps, so you can use data to influence business decisions.
Try a Data Product Accelerator (DPA) for web analytics
Why choose Snowplow for your digital analytics?
Snowplow is a Behavioral Data Platform, built specifically for capturing the smallest details of user behavior and sending this data to your storage destination in a highly-usable format.
We specialise in helping companies create ultra-predictive data sets for AI and advanced analytics. These data sets offer a level of insight that can differentiate you from the competition.
The data hits your warehouse or lake AI- and BI-ready, freeing your data team from endless wrangling and preparation and allowing them to conduct sophisticated experiments to maximize engagement.
Here are some of the features of Snowplow which help you to reengage more customers:
Robust user identifiers
Snowplow provides out-of-the-box user IDs, including session IDs, cookie IDs, and IP addresses.
First-party server-set cookies
These cookies can track customers for up to 400 days (at the time of writing), including Safari users normally blocked by ITP restrictions. Snowplow is actually deployed in your own cloud environment, ensuring this is done in a highly compliant way.
Out-of-the-box e-commerce features
Snowplow provides a set of e-commerce tracking methods and associated JSON schemas that you can use out of the box (or you can create your own custom data structures for your own specific business needs).
130+ metrics tracked automatically
Other metrics which can help you optimize abandoned cart recovery, such as the page the user was on before and after the event happened, the device, location, and other specific user information. Take a look at our modeled data.
Event properties are kept within a single table
This means generating user segments to answer advanced questions is straightforward. These questions include the optimal time to send communications, what counts as an abandoned basket, and which channels to choose.