Redefining web analytics metrics
At the heart of our web analytics are our metrics. These are the ways we measure the performance of our websites or web-based applications, how they are received by our web visitors and whether they are effective, commercially or otherwise.
In this instalment of our series on web analytics, we are going to look at some of the commonly used web analytics metrics and why they don’t always serve their purpose when it comes to understanding user behaviour, both technically and conceptually. We’ll also look at how businesses can go about creating more meaningful metrics to understand their users better.
Most web analysts have used the same common metrics to measure website performance for a number of years. Some of these metrics include:
- Conversion rate
- Bounce rate
- Time on page/Session duration
Most of these metrics are provided to users by a packaged tool like Google Analytics. Most of these out-of-the-box metrics are designed to make it easy to understand if our site is effective at converting users, or they are finding our pages and content engaging – and they are generally all understood to work as follows:
- Conversion rate - the higher the better
- Bounce rate - the lower the better
- Time on page/Session duration - the higher the better
This way of understanding these metrics makes a lot of assumptions on how we believe users interact with our websites. But how valid are these assumptions? And how are these metrics calculated?
Are they appropriate to use to measure the effectiveness of our web marketing and performance of our websites? This post aims to shed light on how tools like Google Analytics serve these metrics and allow analysts to make a better decision on whether to use these out-of-the-box metrics.
Conversion Rate
Conversion rate is designed to indicate how well a website is performing in terms of pushing a user through a desired journey towards a desired conversion – like purchasing a product, signing up for a demo or requesting a call from a sales team.
"92% of users visiting a website are not yet ready to buy"
There is even the specialist field of Conversion Rate Optimization (CRO), which aims at tweaking the website design and user experience to improve its efficacy. A common approach for conversion rate is to try and turn a funnel into a cylinder, removing blockers and issues that make users likely drop off and leave the purchasing journey, and optimizing the experience to make it smoother and easier for users.
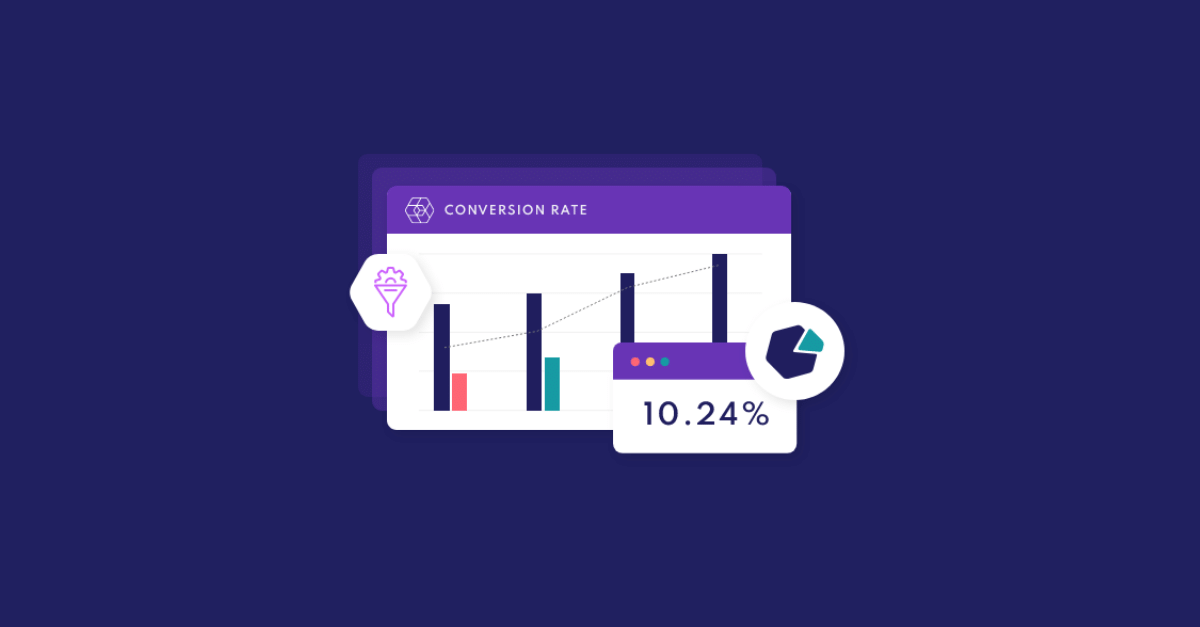
This is a noble aim, and making the user journey a more enjoyable experience for the end user is always a worthwhile effort.
However, the metric itself has problems. The first thing to be aware of is Goodhart’s Law which essentially states that focusing on a single metric like this can have unintended side effects.
According to a recent study, up to 92% of users who visit a website at any given time are not yet ready to buy (depending on the product and the vertical). The vast majority of these users are just browsing to see what’s available at what price, or researching to varying levels to decide what they wish to purchase and where from. Yet, Conversion Rate focuses the mind only on the 8% who are actually ready to buy.
This seems like a misguided approach, as there are numerous ways a brand can add value to a user who is not ready to buy just yet, which may turn them into a customer later on - creating helpful informational content, providing honest comparisons, buying guides, nurturing the user journey until they are ready to purchase - at which point they will be much more likely to visit the site that helped them make their mind up to complete the purchase or conversion, and as a result, more likely to stay a customer and become an advocate.
There are also more concrete technical problems with conversion rate. The biggest issue is that most of the time the conversion rate metric is based on visits or sessions – total number of identified conversions divided by the total number of web visits.
As explained above, the user may be on a long and complex multi-visit user journey, but not quite ready to convert right now. A session based conversion rate would count this visit as a non-converting session, and therefore negatively count towards your conversion rate. But yet, this very user could convert in the future, but the majority of her visits will be discounted, as if they are “bad” sessions, pushing down the conversion rate, and suggesting the website is not performing well.
Conversion rates can be configured to be user based (conversions / unique users), but there are problems with this too. Chief among these is the difficulty in accurately identifying users on the web. Most packaged analytics tools use cookies as their primary identifiers for users visiting the website. Not only does this not reflect users who use multiple browsers or devices, but privacy initiatives such as ITP are limiting cookie’s lifetime to 7 days (and potentially just 1 day), this really plays havoc with the user numbers.
There are ways to identify authenticated users (who login to your site and self-identify) and do so across devices. But this is generally a minority of users, so isn’t a viable option for most businesses.
That isn’t to say that businesses should not concern themselves with their conversion rates, but instead to ensure that they look at the conversion rate metric within the right context, and not be blinded by it. Conversion rates can be useful for visits with a commercial user intent (visits where the landing page is a product page, or PPC traffic from branded keywords for instance) but are less helpful when intent is informational (landing on a content page from organic search) or when the likelihood to convert is low (potentially visits from a mobile device when the product is of a very high value).
Bounce Rate
Bounce rate was once described by Avinash Kaushik as the “I came, I puked, I left” metric back in 2007. It is supposed to signify the amount of your users who landed on your site and quickly decided that your site was not what they were looking for and left instantly. While this is technically true, many analysts and users focus on this metric to measure how a landing page is performing, even though bounce rate has been largely criticized by the wider analytics industry.
Under certain definitions, it is possible for a “bounced” session to actually be a very valuable session.
To understand the issues with bounce rate as a performance measure, we first need to be 100% clear on how bounce rate is calculated. In Google Analytics a “bounced” session is a single interaction session, generally the first page view of the session, and therefore bounce rate is the total number of bounced sessions divided by total sessions.
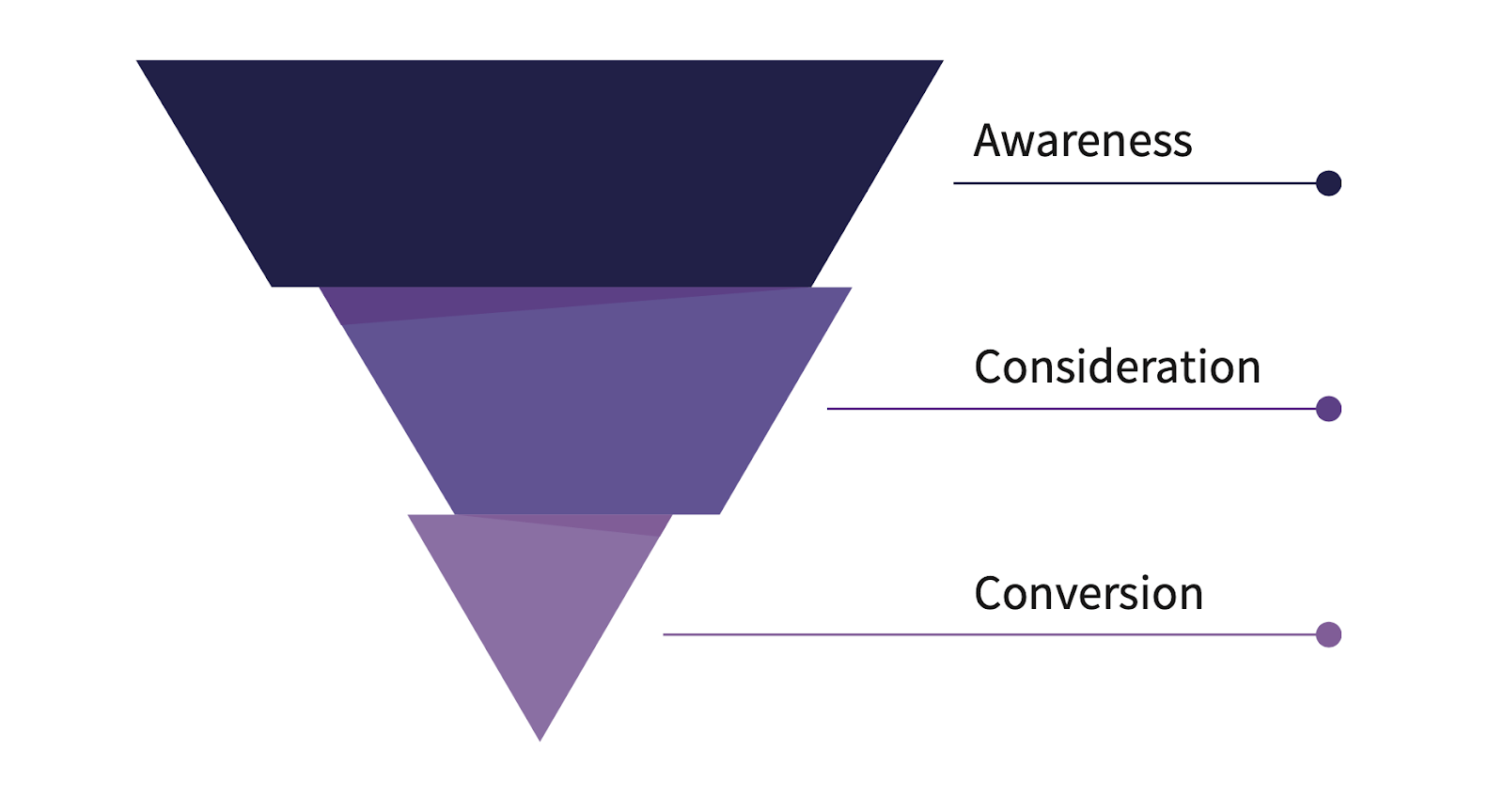
The problems start to occur when we take this as our definition of a bounced session. Under this definition, if there is no other tracking set up to track interactions on the page, it is possible for a “bounced” session to actually be a very valuable session.
A good example is a user landing on a piece of informational content from organic search, perhaps from a “how-to” query such as “how to reverse a commit in git” or “how to make enchiladas”.
They land on the content, spend time on the page, scroll down the page to the end and read the content in full. They may even bookmark the page, or copy the link and send it to their friends or colleagues depending on the exact type of content (not all content is inherently sharable). Having read the content, they’re happy they’ve got what they need, and close the browser tab. This is likely to have been counted as a bounced session, and thus contributes towards increasing the site’s overall bounce rate. And since a higher bounce rate is generally considered to be a bad thing, this is therefore a “bad” visit – whereas in reality this visit was a good visit, as the content answered the user's question and gave them the answer they were looking for.
Another example could be to visit a retail site showing the location and opening times of a physical store. The user gathers all the information they need quickly, and then closes the window. Again, the page has served its purpose perfectly, but still generates a bounced session: therefore, another “bad” visit.
Analysts have seen this happen, and know that a “high” bounce rate is “bad”. As a result, sometimes there is a metric known as “adjusted bounce rate”. This is where the tracking implementation is tweaked in order to bring bounce rate down. For instance, if the user stays on the page for more than 30 seconds, then don’t treat the session as a bounce, even if they then leave. This practice of tweaking or “fixing” the metric is generally not a good idea, as you are not addressing any underlying cause of the metric, rather you are focusing on the metric itself and ignoring the real issue. This means creating content to better fit the user’s intent or optimizing the page for a better experience for the user.
Bounce rate is a useful metric when used appropriately. A good use of bounce rate would be to look across all similar pages (all the content pages within a /blog/ section of a site for example) and compare the bounce rate across all of these pages. If the majority of these pages all have roughly similar bounce rates, but one or 2 have a significantly lower bounce rate, then it’s worth looking into these pages to understand why. This insight could prove valuable when creating future content.
Conversely, if a few pages have a significantly higher bounce rate, then this should be looked to be understood as well. Always make sure you’re doing a fair comparison, you understand the user intent behind those pages and how users got there, and make sure to segment, segment, segment.
Download the full eBook Rethinking modern web analytics
Time on Page/Session Duration
For sites that don’t sell directly online, such as publishers or brochure sites, or sites with a large content section, it can be hard to understand if the content is performing well or accurately understand the value of the content. One way analysts try to measure the value of content and measure its performance is to try to comprehend the engagement that content is creating. Engagement is notoriously difficult to quantify and measure - so a common proxy for engagement is the time a user stays on a specific page or the site as a whole (another common approach is to look at the average number of page views per session).
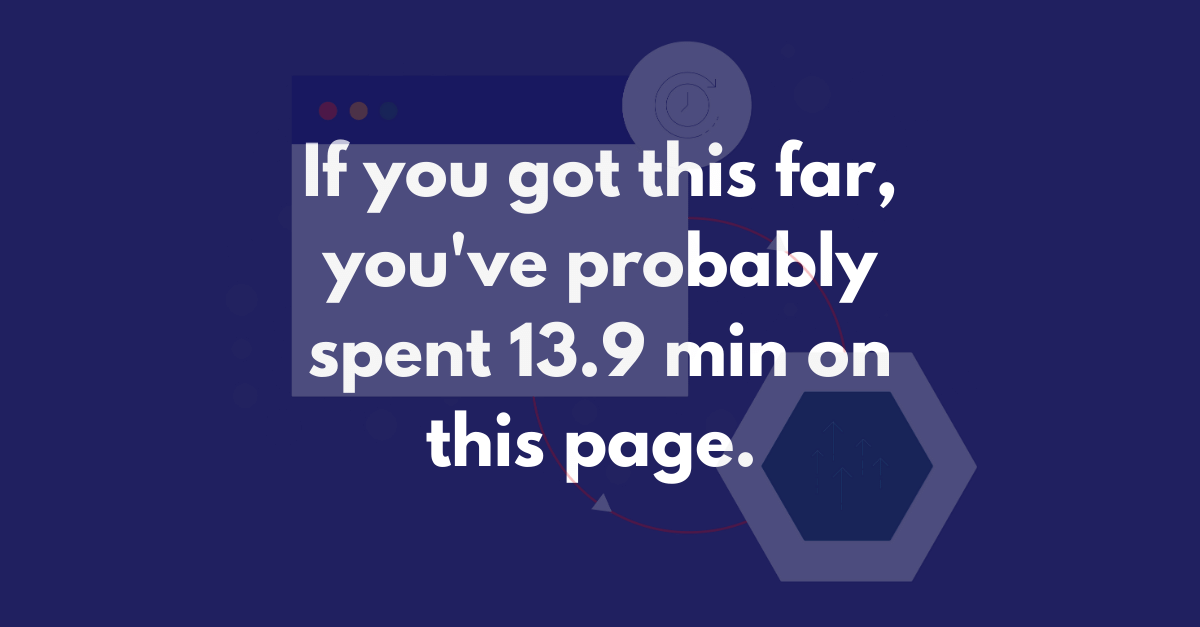
“If there’s one thing a publisher should really understand it’s how much attention their content is receiving. But most media companies really don’t have a handle on it. The most common analytics platforms do such a bad job of measuring it they’re actually worse than useless, so most media companies focus instead on pageviews and reach metrics.”
– Simon Rumble, Digital Analytics Specialist at Australian Broadcasting Corporation (ABC)
However, as you may have guessed, there are both conceptual and technical problems with measuring time on page/site. The first point to consider is this: Does a higher time on site really mean that the users are more engaged with the content or the site? It is true that if a user enjoys the content on a site, that they may spend more time reading the articles and potentially browsing to other articles or pages and reading them.
The problem is that a user who is not enjoying the content or is struggling to use the site could also spend more time on the site. What if the user interface is confusing and the user can’t navigate the site easily? This is likely to mean they will spend longer on the site as well. Or a user who is struggling to read the content because it is too complicated to follow or poorly written? There’s no real way to differentiate between these two very different types of user experience just by examining the time spent on the site.
There are also issues from a technical standpoint. Most tools that measure the time spent on a page or on the site use the difference in timestamps between page views and other page views or other events. The problem with this is that these don’t account for whether the user was actually at their screen. If you view a page, read for 20 seconds and leave your screen to make a coffee for 10 minutes and return before the session times out, it is likely that the tool will assume you’ve spent those 10 minutes looking at the page, even when you weren’t.
Snowplow handles this by using Page Ping events, where the Javascript tracker “pings” the page to see if the user is still active. If not, then this time is not taken into account when calculating how long was spent on the page.
“With page pings from Snowplow we have a very precise way of measuring engagement on our articles. This is something we simply couldn’t do before with Google Analytics. I think this is one of the most interesting metrics we’ll see in terms of media analytics.”
– Aurelien Rayer, Head of Data at Welcome to the Jungle
Another issue specific to Google Analytics is how GA handles Exit Pages. An exit page is the last page in a user’s session. Since GA generally calculates the time on a page as the duration between 2 page views, this cannot be calculated for an exit page as there is no subsequent page view - and it then sets the time spent on that page to 0 seconds which is clearly not reflective of what has actually happened. However, despite the fact GA does not know how long a user spends on their session’s exit page but sets it to 0 seconds anyway, the exit page view is still included in the average time on page calculation (total time spent on the site / total page views). This method of handling time on page for an exit page messes with both the numerator and denominator in the calculation, causing the metric to be fundamentally flawed.
What metric should web analysts focus on?
If the standard metrics that are provided by packaged analytics tools have issues, what should we use instead?
The first thing to say is that these metrics aren’t always the wrong thing to use. It’s just important to understand how they are measured and calculated, so that given your unique case you are able to make a call as to whether these metrics are appropriate or not.
Given this, are there alternatives to these common metrics that can be used in their place? Or different applications of these metrics that will make them more meaningful?
Session and user based conversion rates all suffer from the somewhat flimsy definition of “sessions” (a collection of hits with a timeout window, as well as other factors) and “users” (a unique cookie ID, unique to a browser/device combination). A way to look at making conversion rate more meaningful is to use it in the context of other important events (sometimes called micro conversions).
This is common when looking at “funnels” on site - what percentage of users who perform action X then go on to perform action Y. This is more concrete than relying on artificial concepts like sessions or users, and as long as all important micro conversions are tracked on your site or in your product, these kinds of “this-then-that” analysis can be performed straightforwardly.
Time on page/site is problematic as it is hard to differentiate between different user experiences from a single value. Utilising a user engagement metric that actually detects the time actually spent at the screen (using a heartbeat mechanism or similar) helps only measure time users have spent interacting with your features or content.
While this helps make the metric somewhat more reliable, the most important thing to change is your mindset when analyzing the data. Make sure to take into account things such as how long the content is, what the user intent was when they landed on the page (whether from a search engine, a social media post, a referring site or an ad), any multimedia content (video or audio etc) which might change the user’s behaviour etc. Once these factors are considered, you are in a better position to interpret what a particular metric might be indicating.
The ultimate aim is to create metrics that are completely custom to your site or product, and limit your use of “standard” metrics to all but the most top level of analyses. This takes a deep level of understanding of your site, your users and their user journeys. But once you have these higher value, customised metrics that are much more meaningful, drawing insights from your data becomes much easier.
This is an 8-part series
Click below to navigate to the next chapter:
- Chapter 1 The state of web analytics in 2021
- Chapter 2 Privacy updates, ad blockers, and the need for 1st party tracking
- Chapter 3 Building a web analytics stack: packaged vs modular
- Chapter 4 The best-in-class-tools for web analytics
- Chapter 5 Redefining web analytics metrics
- Chapter 6 Data modeling for web analytics
- Chapter 7 Snowplow for web analytics
- Chapter 8 How Welcome to the Jungle took ownership of their web data with Snowplow