5 Key Takeaways From Big Data LDN 2024
Once again, the Snowplow team had the pleasure of exhibiting and speaking at Big Data LDN - the UK’s premier data, analytics, and AI event. And it certainly didn’t disappoint. Whether you work for a startup or a global enterprise, the event was a fantastic opportunity to gain valuable knowledge from expert speakers and success stories from the world’s most recognizable brands. Here, we share our five key takeaways from this year’s event.

- Garbage in. Garbage Out.
- Build Data Flywheels to Gain a Competitive Advantage
- Lessons From Supporting Modern Data Lake Formats
- The Importance of a Data-Driven Culture
- The Rise of AI Agents
1. Garbage in. Garbage Out.
One critical step often gets overlooked when adopting AI tools: ensuring you have high quality data.
Over the two day conference, we heard numerous vendors and speakers emphasize the adage “garbage in, garbage out” when it comes to AI applications. Before diving into AI, your business must prioritize cleaning, standardizing, and validating your data to avoid misleading or irrelevant results. AI-ready data is becoming foundational for any business looking to unlock its potential.
Yali Sassoon, CTO and Co-founder of Snowplow reinforced this point in his talk: “If your organization is trying to use AI to drive competitive advantage, really the only place you can do that is in data and this really needs to be proprietary data.” This is a perspective that is echoed around the industry. In fact, researchers at Gartner note that 70% of AI programs today falter due to poor data quality.
“Many businesses misconceive AI as just code, when in fact, the AI program is data,” says Susan Laine, Chief Field Technologist at Quest Solutions. As exciting as AI is, you can’t skip the vital step of ensuring you have good trusted data.
WATCH: Build Confidence in Your Digital Analytics Data Quality with Snowplow
2. Build Data Flywheels to Gain a Competitive Advantage
To overcome the garbage in, garbage out challenge, data flywheels are a great place to start.
A data flywheel is a self-reinforcing cycle where your proprietary data powers AI applications. Once deployed, these applications generate new data from their interactions and outcomes. This data is then fed back into the system, continuously improving the AI’s performance and creating a sustained competitive advantage.
During his talk at Big Data LDN, Snowplow’s Yali Sassoon explained how companies like NVIDIA are using data flywheels to form a huge competitive advantage:
“NVIDIA feeds years of GPU design data into LLMs, which then design new chips. Each new design generates more data, continuously improving the LLMs. This flywheel effect makes it nearly impossible for competitors to catch up, as NVIDIA's AI gets smarter with every iteration.”
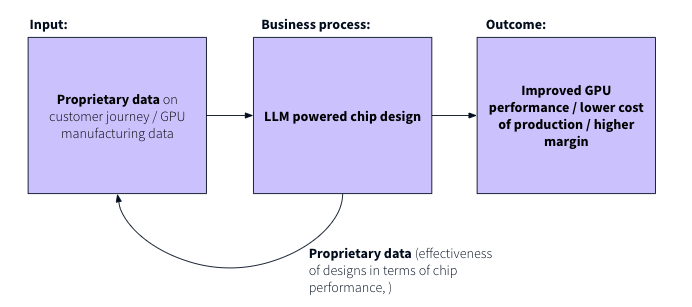
Key components for building effective data flywheels include:
- Proprietary, AI-ready data
- Strong data governance
- Efficient feature pipelines
- Robust technology architecture for data collection
- Ability to track AI predictions and their outcomes
Yali concluded, "We need to go beyond just building our initial proprietary data set. For every algorithm we have running in production, we must be able to track precisely what predictions it's making and how successful those predictions are. This ongoing measurement and feedback loop is crucial for continuously improving our AI systems and maintaining our competitive edge."
LINKEDIN LIVE: Building a Personalization Flywheel from AI-Ready Behavioral Data
3. Lessons From Supporting Modern Data Lake Formats

Flywheels and AI-ready data are only good if you can store your data in a scalable and cost-effective way. Data lakes and lakehouse architectures are having a resurgence as of late given their role in supporting AI workloads. On day one of the conference, Snowplow’s Jordan Peck packed out the Data Engineering Theatre to share his lessons learned from supporting modern data lake formats at Snowplow. They include:
- Choose your metadata catalog wisely. Even on the same cloud, Unity Catalog, AWS Glue, and Hive Metastore play by different rules. Check their authorization methods and storage formats.
- Plan your partitioning strategy carefully. If you need flexibility, consider Iceberg. This supports partition evolution, boosting your query performance and data management options.
- Watch out for high-volume write hiccups. With Delta, for instance, you might face duplicate data due to full metadata commit log scans. Tune your write options accordingly.
- Mind the data type gap. If you’re using Iceberg with Snowflake, be prepared to adjust your dbt code. Iceberg loves structured objects, while Snowflake prefers variant columns for semi-structured data.
- Speak the right SQL dialect. Snowflake, Databricks, and Athena each have their own lingo. Adapt your transformation code to fit.
As Jordan mentioned, choosing the right setup can feel like ‘playing a slot machine.’ But armed with these insights, you’re on your way to winning the data lake game.
PRODUCT FEATURE: Snowplow’s Lake Loader
4. The Importance of a Data-Driven Culture
Your data and AI success is dependent on your organization’s culture. As Hilda Sadek from Conde Nast aptly put it while speaking at the event, “Data culture is characterized by recovering data into the operations mindset and identity of an organization providing everyone with the insights they need to make a data informed decision.”
Multiple sessions at Big Data LDN explored the challenges of building, scaling, and maintaining a data-driven culture. From Lloyds Banking Group’s strategies for scaling a data culture to Yusen Logistics’ transformation journey, the conference emphasized that culture drives outcomes.
Peter Laflin from Morrisons highlighted the human element: "It's the people, as well as the technology, that has enabled us to get the real time data that's making such a big difference for our customers' experience."
While technology is important in today’s data rich environment, cultivating a robust people-centric data culture is essential for your organization to be successful.
5. The Rise of AI Agents
AI agents are seen as the next frontier in artificial intelligence. This emerging trend was reflected in several keynotes such as "Data Agents vs Data Chatbots" and "How To Build an Enterprise AI Applications With Multi Agent Rag Systems (MARS),"
But what are AI agents exactly? In plain terms, they're advanced AI systems that autonomously interact with their environment. They make complex decisions. They learn from feedback to perform tasks that traditionally require human expertise such as designing GPUs or creating content.
Snowplow’s Yali Sassoon also touched on the emerging role of AI agents. He referenced how NVIDIA is using agentic applications to revolutionize how it approaches complex design tasks.
AI agents form a part of NVIDIA's data flywheel. They're powered by proprietary data - including design history, test results, and sales information - allowing them to design new GPUs.
But challenges remain with these technologies. Seamless integration, user-friendliness, and trustworthiness are crucial for widespread adoption. There are also ethical considerations, including data privacy and algorithmic biases that must be addressed.
Having said that, the message from the event is that AI agents are no longer optional. They’re becoming necessities for sustaining a competitive edge. At Snowplow, we’re building Customer Data Infrastructure that’s equipped to support a martech future driven by these AI agents (as Scott Brinker recently pointed out).
Charting the Course for Data-Driven Success
So that’s it for Big Data LDN 2024 and the messages are clear: high-quality proprietary data has to be the foundation of your AI initiatives. From data flywheels to AI agents, each takeaway underscores the same message: organizations must prioritize data quality, culture, and innovative approaches to remain competitive.
Keeping aligned to these principles will be fundamental as we progress into the AI-driven world. Good luck and we’ll see you next year!
Learn How to Make Your Organization AI-ready
If you’d like to learn more about how Snowplow’s Customer Data Infrastructure (CDI) can fuel AI for your business, request a demo with us here: https://www.snowplow.io/get-started/book-a-demo-of-snowplow-bdp
During the demo, our experts will show you how Snowplow’s CDI can empower your organization to collect, manage, and utilize high-quality behavioral data to power advanced use cases, all while ensuring data security and compliance.