Calculating customer value allows you to put your focus on the customers who use your products and services the most, improving the efficiency of your product development and marketing campaigns.
Section 1: A broad overview of the benefits and challenges of a warehouse-first approach to customer lifetime value
Section 2: A technical guide to calculating customer lifetime value
Section 1
What is customer lifetime value?
The idea behind customer lifetime value is simple: it is the total amount of value derived by a business from a customer over the customer’s complete lifetime engaging with the business / product / company.
Calculating and maximizing a customer’s lifetime value contrasts with other approaches to increasing value e.g.:
- Optimizing basket size: where we optimize a specific “shopping visit”, rather than all the shopping visits performed by the customer. Amazon Prime is a great example of an initiative that reduces basket size (by encouraging members to place single item orders) but grows customer value nevertheless (by driving increased number of purchases by customer).
- Optimizing occupancy: where we optimize the revenue / profit per room or per seat rather than per customer.
- Optimizing sell-through rates: where we optimize either the fraction of something that is sold (where we do not control the total volume available e.g. ad inventory), or the volume produced to maximize profit (e.g. physical CDs: the more we cut the more we can sell, but the more are likely to remain unsold and therefore a net cost to the business)
Why is customer lifetime value important?
Maximizing customer lifetime value is generally the most sustainable way to drive value growth for any consumer-facing business, because:
- A minority of customers often account for the majority of profit. The best customers might be brand loyal, not “shop around”, rich or have other features that mean they behave differently from the majority of customers.
- Cost of acquisition is trending upwards. As a result, it is often more cost effective to spend money retaining existing customer rather than acquiring new customers. In addition, when acquiring new customers, the amount that should be spent varies widely depending on the type of customer acquired.
- It forces the business to take a long term approach to value creation rather than e.g. short term promotions that maximize revenue on a particular shopping trip, or particular product line, at the expense of eroding brand value.
- It emphasizes customer loyalty, which has repeatedly been shown to be an intangible asset that successful companies can monetize over long periods of time.
- It points to bigger opportunities for value creation (e.g. moving into new markets / complimentary services) rather than smaller opportunities (e.g. produce less of this particular CD because we do not think it will sell well in this market). In general, that is because the upper limit to the value you can generate from each customer will be much higher than the upper limit for a basket of goods or bed in a hotel, for example.
Maximizing customer lifetime value is only possible if you can measure it (and therefore track improvements over time). So measuring customer lifetime value is critical to driving long term growth for consumer-facing businesses.
Where is customer lifetime value used?
Customer lifetime value is used to support a range of business functions. Two, however, stand out in particular:
- Driving your acquisition strategy. Tailor spend per channel based on the expected customer lifetime value of customers acquired from those channels.
- Improving your customer relationship management (i.e. grow loyalty, reduce churn). Personalise marketing, communication and promotions to the existing customer base to maximize their loyalty and customer value. (For example, tailor spend to cultivate loyalty in the most valuable customers.)
The limitations of traditional web analytics tools when calculating customer lifetime value
Traditionally, web analytics tools have sucked at enabling analysts to calculate customer lifetime value:
- They don’t make it easy to capture value outside of transaction-based businesses, e.g. ecommerce
- They don’t make it easy (or often possible) to slice all historical data by customer. (Because they typically only expose session-level data.)
- They don’t integrate with other systems used in other channels, to enable a holistic view of customer behavior and value across online and offline channels
- They don’t provide the granular data required to build sophisticated models to forecast customer value going forwards
Fortunately, Snowplow addresses the above limitations, enabling analysts to calculate customer lifetime value as detailed below.
Section 2
How to calculate customer lifetime value with Snowplow
There are different levels of sophistication to calculate customer lifetime value. The diagram below illustrates some different approaches:
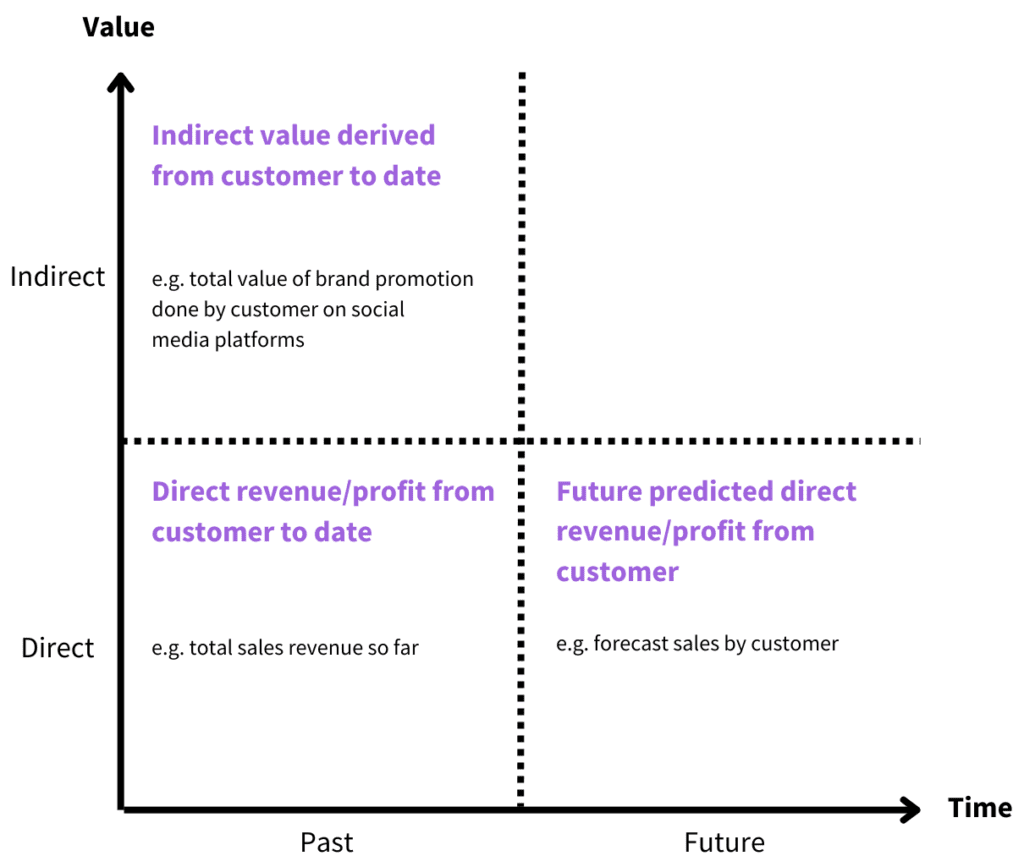
We can distinguish different levels of sophistication when measuring customer lifetime value:
1. The simplest approach to calculating CLV
When starting out, companies can choose to define customer lifetime value as the total revenue earned from this customer to date. This provides a past-facing metric on which to base decisions related to that customer or group of customers.
2. A more sophisticated approach to CLV
Once a basic – past facing – understanding of CLV is achieved, teams can start to estimate the total lifetime value of the customer (past and future) based on the customer’s historical data. AI and ML can be particularly beneficial when identifying patterns here, although BI approaches are also possible.
For example, a mobile telco business may assume an average length of contract for each customer, and project the expected revenue earned from each customer based on their predicted contract length. This figure could then be included as part of the total Customer Lifetime Value.
3. Additional advanced strategies
a) We can be even more sophisticated about how we model future revenue, for example, by using different figures for different customers based on what customer segment they belong to.
b) Further to projecting revenues going forwards, we might want to discount future revenue to net present value in our calculation.
c) Instead of measuring the revenue earned for each customer, we might want to look at profit. This prevents a business focusing unnecessarily on high revenue but unprofitable customer segments.
d) Instead of looking at the revenue / profit directly attributable to each customer, we might want to value the other activities that a customer engages with that create value but do not directly result in revenue. This is important for social networks / community sites where users contribute content, for example.
e) Which approach you adopt will depend on your own circumstances, data quality and customer understanding: a cruder approach will often suffice and a more sophisticated approach may be misleading if there isn’t the customer understanding in place to justify the assumptions an analyst uses in predicting future revenue / profits per customer.
Why choose Snowplow for measuring customer lifetime value?
Snowplow is a Behavioral Data Platform, built specifically for capturing the smallest details of user behavior and sending this data to your storage destination in a highly-usable format.
We specialise in helping companies create ultra-predictive data sets for AI and advanced analytics. These data sets offer a level of insight that can differentiate you from the competition.
The data hits your warehouse or lake AI- and BI-ready, freeing your data team from endless wrangling and preparation and allowing them to conduct sophisticated experiments to maximize engagement.
Here are some of the features Snowplow offers:
Robust user identifiers
Snowplow provides out-of-the-box user IDs, including session IDs, cookie IDs, and IP addresses.
First-party server-set cookies
These cookies can track customers for up to 400 days (at the time of writing), including Safari users normally blocked by ITP restrictions. Snowplow is actually deployed in your own cloud environment, ensuring this is done in a highly compliant way.
Out-of-the-box e-commerce features
Snowplow provides a set of e-commerce tracking methods and associated JSON schemas that you can use out of the box (or you can create your own custom data structures for your own specific business needs).
130+ metrics tracked automatically
Other metrics which can help you optimize abandoned cart recovery, such as the page the user was on before and after the event happened, the device, location, and other specific user information. Take a look at our modeled data.
Event properties are kept within a single table
This means generating user segments to answer advanced questions is straightforward. These questions include the optimal time to send communications, what counts as an abandoned basket, and which channels to choose.