Define & Track
Behavioral Data
Generate rich, first-party event data from across the complex customer journey and load it into your data platform.
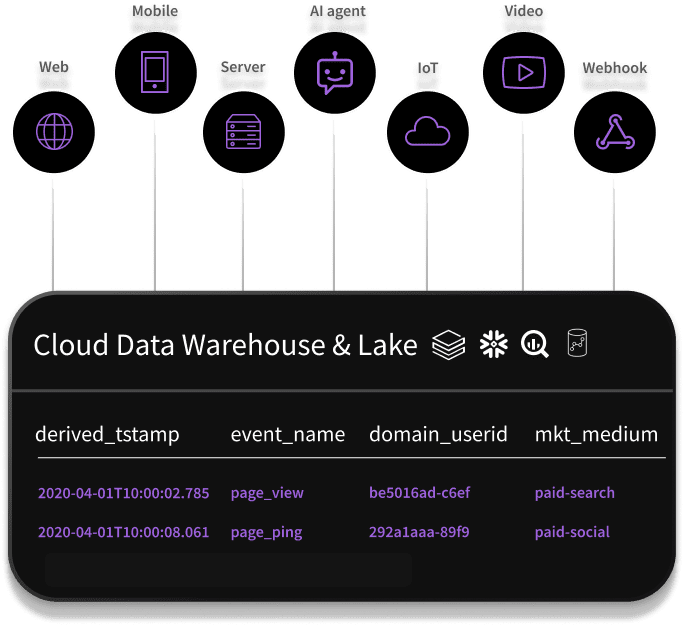
Collect Behavioral Data Across Your Digital Platforms
Snowplow provides the most comprehensive set of trackers and webhooks to collect customer behavior across all touchpoints, including AI agents, wearables, IoT, and video. Collect the most comprehensive, validated behavior data designed specifically for your warehouse or lake.
Load data from 35+ dedicated first-party trackers and webhooks
With 35+ dedicated first-party trackers and web hooks you can track events and entities and deliver a single unified event stream to warehouse, lake or to a real-time stream.
Understand the customer intent using events and entities
Snowplow’s unique approach enables you to track events and the context an event took place with entities, offering the most accurate insights for predicting and understanding customer actions.
Collect a complete view with persistent device tracking
Track customer behaviour for up to 2 years and with Snowplow’s ID service you can strengthen tracking capabilities where browser limitations such as Intelligent Tracking Prevention (ITP) are enabled.
Leverage Rich Behavioral Data to Understand, Predict, and Influence Your Customers
Capture an expansive and rich event data to power accurate model predictions or understand customer behavior.
Out-of-the-box and
custom tracking
Every Snowplow tracker allows you to automatically track a variety of out-of-the-box events and entities, or you can create your own custom tracking. From scroll depth to engagement in ads in your media player, we’ve got you covered.
130+ properties captured with every event
Collect 130+ properties with every event providing a large number of features to create accurate model predictions or for in-depth analysis.
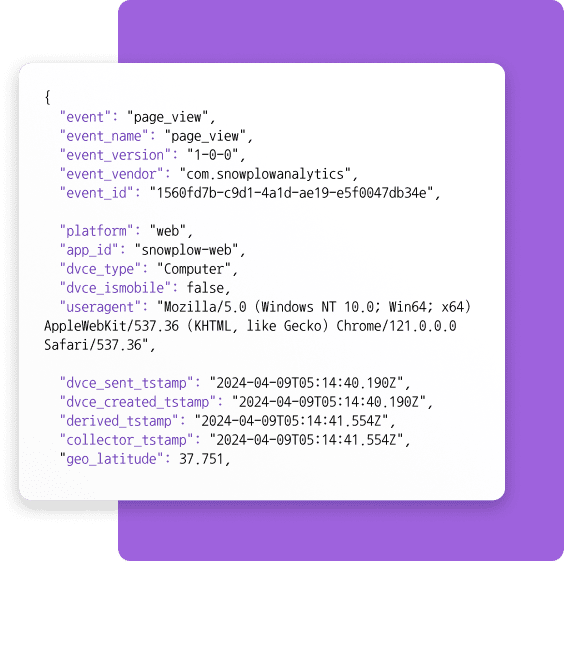
Ensure data quality at point of collection
Snowplow ensures data quality at the point of collection, guaranteeing that only accurate and reliable data powers your insights and data products.
Upfront validation
Control the quality of your data via dynamic schema. Define and enforce policy on how data is created, processed, and delivered to your warehouse or lake.
Real-time data quality monitoring
Monitor data quality metrics and performance indicators in real-time in platform or via API.
Load Behavioral Data in Real-time to Your Data Warehouse or Lake of Choice
Snowplow unifies and delivers all your tracked events and their attributes in a single, unified event table in your warehouse or data lake of choice. With a single structured table avoid complex joins and scale your use of behavioral data with ease.


Choose Where Snowplow
is Deployed
Choose how you want to deploy Snowplow, use Private Managed Cloud for full ownership or control or let Snowplow do the heavy lifting with BDP Cloud.
Private Managed Cloud
Deploy Snowplow in your own cloud providing full ownership and control over your customer data. Configure your deployment with additional infrastructure security to meet your needs.
Hosted by Snowplow
Let Snowplow host and manage the deployment of your data pipeline with data streamed into your warehouse or lake using BDP Cloud.